Allergy algorithm to increase pre-test probability of allergic disease
Introduction
The interpretation of allergy diagnostics results has become more and more complex, due to the recent diagnostic developments. In the past decades, scientific research in allergy diagnostics has increased tremendously, both on immunological and diagnostic level (1). Where initially allergen extracts were used to determine allergy diseases, nowadays, one is able to determine individual proteins [molecular components (MCs)] for a more specific analysis of these diseases. For example, while formerly an allergic reaction was attributed to dust, it was later discovered that dust mite was the cause of complaints. These days, different allergenic MCs (proteins) of dust mite can be analysed and each MC can be related to varying degrees of reaction. Analysis of more than hundred MCs is already available in routine allergy diagnostics (1).
Another aspect of this development is that some components have no clinical importance or give mild reactions while others may cause severe anaphylactic systemic reactions. Furthermore, processing of some allergens may change the importance of the allergen. For instance, a protein Gly m 4 in soy i.e., soy milk can give a severe allergic reaction, but when soy is processed into flour, another protein (Gly m 5) is responsible for the severe allergic reaction. In screening panels soy (bean) as in soy flour is tested and soy as in soy milk is not tested. This aspect is not familiar to all clinicians/general practitioners and may cause misinterpretation. The lack of knowledge of how these proteins react under different conditions can give rise to inadequate testing, unnecessary costs for allergy diagnostics work-up and misdiagnosis. Based on wrong screening and interpretation of results, unnecessary testing (skin prick test or oral food challenge) by the allergist may follow, as well as consult with a dietitian resulting in lifelong dietary adjustments.
Recently, a framework for the interpretation of IgE sensitization tests has been proposed by the taskforce of EAACI to reassess the use of IgE sensitization tests (skin prick tests and/or serum-specific IgE). The taskforce involves a wide range of clinicians, scientists and patient groups (2). They underline the importance of approaches which include pre-test probabilities in the interpretation of results especially at individual patient level. These types of integral approaches do not exist yet and should be developed.
Some commercially available software packages automatically generate explanatory texts in order to facilitate the interpretation of results. There are in general two limitations of the existing software packages. One limitation is that the packages do not combine the clinical symptoms with targeted laboratory analysis and results in order to contribute to the improvement of the pre-test probability, in general. Other are focused on only one or two symptoms. For example, many efforts were put in the development of software for allergic rhinitis and asthma (3,4). In 2016, a smartphone app was developed to help in diagnostics of pollen allergy (5). However, there is no published approach developed which includes also other aspects/symptoms of IgE-mediated allergy, like food allergy, anaphylaxis, etc.
The aim of the study was (I) to develop an algorithm that would connect clinical symptoms with information regarding the possible sensitization, automatically generate optimal testing and interpretative comments; and (II) make a preliminary analysis of its implementation.
Methods
Development of the algorithm
In 2014, we developed an algorithm useful in IgE-mediated allergy disease based on clinical symptoms alongside information regarding the possible sensitization of the patient (Figure 1). The algorithm automatically generates tests as well as interpretative comments. The comments are based on recent guidelines on inhalation and food allergy (3,6-8). We aimed to help clinicians to differentiate severe from non-severe cases and advise them if further consultation by an allergy specialist is recommended. Furthermore, whether immunotherapy as in case of inhalation allergy might be an appropriate treatment.
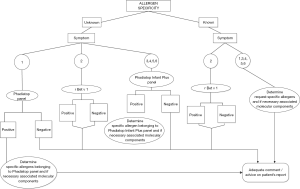
The algorithm is based on clinical symptoms and known allergen specificity. The clinical symptoms are classified into six categories: (I) rhinoconjunctivitis/asthma; (II) oral allergy syndrome (OAS); (III) acute urticaria/angioedema; (IV) acute eczema/atopic dermatitis; (V) anaphylactic shock; (VI) a combination of these symptoms (Figure 1). These categories are in line with categories as recently proposed (2). When ordering allergy testing in our hospital information system clinicians must indicate a symptom category and choose whether the allergy specificity is known or unknown. Based on these criteria specific allergy tests are generated and accordingly, testing is performed. The information on symptom category and allergy specificity is recorded as separated variables. If the allergy specificity is unknown, automatically the mixtures of allergen extracts, either Phadiatop (inhalation screening) or Phadiatop Infant plus (inhalation/food screening) are generated depending on the symptoms. When allergy specificity is known, all requested allergens are analysed directly without performing an initial screening (Figure 1). If allergy specificity is unknown, and screening with the mixture of allergen extracts is tested positive, the relevant extracts are tested automatically. Accordingly, if an extract is found positive, the associated MC(s) are tested. This reflex-testing is restricted to the MCs Ara h’s, Cor a’s, Gal d 1 and Bos d 8, respectively related to most frequently tested food allergens peanut, hazelnut, egg and cow’s milk. In case of positive inhalation screening, comparable reflex testing will be done and on request the appropriate, MCs are tested. When tree pollens, grass pollens and/or house dust mite allergy extracts are positive, upon advice, patients can qualify for immunotherapy in early onset of allergy disease. All other available MCs will be analysed only in agreement with or by recommendation of the allergy specialist.
In summary, the laboratory results are accompanied by appropriate commentary relating risk of eating certain foods, raw or processed, to symptoms. The commentary is also providing clinicians with advice to consider immunotherapy as an option according to the ARIA recommendations (3,8-10). The programming of this algorithm was within the framework of our laboratory information system (TECHNIDATA Benelux B.V. medical software and later on GLIMS version 9.5/MIPS), but can be transposed to any other laboratory information system.
Case examples
As an illustration of the mechanism of this algorithm, consider an example of a patient (>5 years of age) with rhinoconjunctivitis complaints with unknown allergen specificity consulting ear-nose-throat (E.N.T) specialist. When requesting allergy diagnostics, the clinician chooses symptom category rhinoconjunctivitis/asthma and unknown allergen specificity. This combination of variables automatically generates a Phadiatop screening and all (other) allergy-related test requests are cancelled. If the result is negative (Phadiatop <0.35 kU/L) the explanatory comment: “If Phadiatop screening is negative, allergic rhinoconjunctivitis/asthma is unlikely” is added as a commentary text to the results of the allergy testing report.
Furthermore, a complex example, if a patient is tested positive for hazelnut-extract (>0.35 kU/L) the associated MCs Cor a 1 (PR-10) and Cor a 8 (LTP) are automatically analysed. The comment generated on the patient report is as follows: “Cor a 8 positivity (>0.35 kU/L): risk of severe clinical reaction. Cor a 1 positivity (>0.35 kU/L): none to mild reaction like OAS (in which symptoms are manifested as irritation in the mouth, swelling of lips, mouth and/or throat)”. However, when Cor a 1 and Cor a 8 are negative, automatically Cor a 9 (storage) and Cor a 14 (storage) are analysed and upon positive result the following text is generated: “Cor a 9 and/or Cor a 14 positivity: risk of severe clinical reaction”.
Retrospective analysis of the effect of introduction of the algorithm
The algorithm was introduced in our hospital in 2014, with an introduction period from September to December 2014. Preliminary analysis of the implementation of the algorithm was performed through retrospective analysis of patient data from the departments of allergy, paediatrics, dermatology and E.N.T. This retrospective analysis consisted of three parts: (I) comparison of allergy diagnostic patterns before introduction to after introduction of algorithm (Jan/Feb 2014 to Jan/Feb 2015); (II) distribution of symptoms for whole 2015; and (III) the frequencies of positive extracts were related to the frequencies of positive MCs in whole 2015.
Biochemical testing
Blood was collected by venipuncture. The analysis consisted of determining tIgE, sIgE of extracts and sIgE of food or inhalation MCs. The analysis of the tIgE and sIgE with the ImmunoCAP Components (Thermofisher Scientific/Phadia Uppsala, Sweden) were performed according to the manufacturer’s instructions. The ImmunoCAP is a solid phase enzyme-immunological assay for quantitative measurement of tIgE and different types of sIgE. The solid phase is a cap coated with antigen (allergen). The test principle is that antibodies present in serum will react with the coated allergen and after incubation with a fluorescence and enzyme-labelled antibody, the fluorescence is measured. The measured fluorescence response is a value for the quantity of antibodies present in serum [source: Directions for use ImmunoCAP® tIgE (2014 March 8th)]. Reproducibility and linearity were checked for sIgE. As a model, allergen of venom MC Api m 1 (I208) was used. Reproducibility was within the manufacturer specifications, for sIgE <7.8% (concentration range, 4.9–16.7 kUA/L). For linearity (Deming) regression yielded a slope (95% CI) of 1.010 (0.944–1.29) and an intercept (95% CI) of 0.17 (−0.94–1.29). Correlation coefficient (r) was 0.997.
Measuring ranges were 2–5,000 kU/L and 0–100 kUA/L respectively for tIgE and sIgE. Reference values were according to manufacturer recommendations: <0.35 kUA/L and <100 kUA/L, respectively for sIgE and tIgE. They however show age-dependency, and therefore in children age-dependent values were used. One IU IgE is equivalent to 2.42 ng of IgE according to NCCLS guideline I/LA20-A2.
Statement of ethics approval
The Executive Committee of the Medical Ethical Committee, has reviewed on February, 22st, 2017 and Board of Directors approved the Research Protocol (Protocol T2017-14).
Results
Comparison of allergy diagnostics patterns before introduction to after introduction of the algorithm (Jan/Feb 2014 to Jan/Feb 2015)
Patient data from the mentioned departments were retrospectively reviewed before (Jan/Feb 2014) and after introduction of the algorithm (Jan/Feb 2015) as presented in Figure 2.
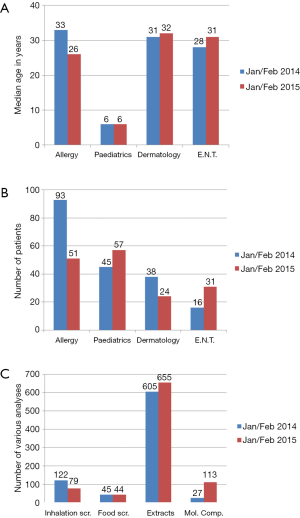
Although the number of patients in 2015 decreased for the allergy and dermatology departments, and increased for the paediatric and E.N.T. departments, no big difference in age distribution, at which patients presented, was found. Gender distribution was skewed, slightly more females. The distribution of gender in Jan/Feb of 2014 was comparable to Jan/Feb 2015 (both 56% females).
The number of inhalation screenings in the period in 2015 decreased with 35% as compared to the same period in 2014, whereas the number of food screenings remained unchanged. The number of MCs requested increased significant, as a result of the different workflow and increasing availability of MCs. So for example, a positive peanut extract screening leads to reflex testing of three components (Ara h 2, Ara h 3 and Ara h 8), while positive hazelnut screening leads to a two-step reflex testing, combination of Cor a 1 and Cor a 8, if both negative, Cor a 9 and Cor a 14 are tested. Whereas in 2014 before introduction of the algorithm, peanut or hazelnut positive screening led to testing of usually one MC, respectively Ara h 2 or Cor a 1, exclusively upon advice of allergy specialist. Analysis of the workflow in relation to positivity of different combinations of allergen extracts is elaborated in section 3 of this retrospective analysis.
Distribution of requested symptoms in whole 2015
As data analysis of Jan/Feb 2015 showed that OAS is not requested, we analysed the whole of 2015 to see whether this changed with the longer application of the algorithm. Despite some small changes in symptom distribution, the most striking feature of the analysis is the lack of recognition of OAS as a symptom. OAS manifests itself as irritation, itching and/or swelling of the mouth, lips and/or throat, generally not giving rise to severe reactions. However, as those symptoms may be experienced as burdensome by patients, its presentation may be misleading. OAS symptoms are mostly, but not exclusively, caused by PR-10 related proteins. The PR-10 MC of Birch, r Bet v1, abundant in Birch extract is important in allergy cross-reactivity and may explain multiple extracts positivity. When r Bet v 1 is positive all other PR-10 related MCs from other allergen sources are likely to be positive. This was shown by several studies, e.g., in one of them the immunotherapy with purified r Bet v 1 showed that immunotherapy with r Bet v1 can help resolve food related problems in OAS caused by PR-10 proteins (11). To help clinicians with the interpretation of a positive result of r Bet v 1 the following explanatory text is generated: “There is a relevant sensitization against the most common protein in tree pollen (Bet v 1). This can cross react with similar proteins in terms of structure and function (PR-10) mainly in pit fruits, stone fruits, nuts and soy milk. Not all products give complaints. Most fruits and vegetables are often tolerated after heating or processing. Known is the cross-reactivity between birch and fruits from the family of rosacea (e.g., apple, peach, kiwi, tomato, nuts)”. Nevertheless, positivity of r Bet v 1 does not rule out a severe reaction on e.g., peanut or hazelnut, as birch allergy can co-exist in combination with for example peanut or hazelnut allergy. As Ara h 8 (peanut) and Cor a 1(hazelnut) are both PR-10 proteins and cross react with r Bet v1, while Ara h 9 (peanut) and Cor a 8 (hazelnut) both LTP proteins can be responsible for severe allergic reactions. LTP’s are heat resistant proteins and still cause reaction after food is processed.
In our region, OAS is occasionally caused by profilins, and in some cases thermo- and gastro-stable molecules. So, in patients with persistent complaints of OAS and negative for r Bet v 1 a consultation with an allergy specialist for further analysis is advised.
Analysis of frequencies of positive extracts in relation to MCs in patients with unknown allergy specificity (year 2015)
As previously discussed, the PR-10 MC of Birch, r Bet v 1, is important in allergy cross-reactivity and may explain multiple extracts positivity. As we assumed that the use of Birch (r Bet v 1) screening as a starting point, in some cases may result in further redundancy of allergy screening the relationship between screening panel (Phadiatop Infant Plus) and birch positivity was examined. In addition, we analysed the frequencies of positive MCs which followed through the algorithm.
In 2015, in total 207 Phadiatop Infant Plus mixture screenings (consisting of Phadiatop Infant and so called Plus or FX77 screening) were initiated based on the algorithm (symptoms 3, 4, 5, 6 in Figure 1). Out of 207, 116 (56%) were positive for Phadiatop Infant and 41 (20%) were positive for FX77. The remaining samples were negative (24%). The number of Phadiatop Infant Plus mixture screenings in the period Jan/Feb 2015 was comparable to the period March to December 2015.
In the whole of 2015, Phadiatop Infant mixture positive sera were positive in 28 (24%) cases for peanut, in 33 (28%) for cow’s milk, in 24 (21%) for egg and in 31 (27%) for Birch. FX77 mixture positive sera were in 35 (70%) cases positive for hazelnut, suggesting that positivity to FX77 is most frequently due to hazelnut in this analysed population.
The distribution of tested components was as follows: Cor a 1 (PR-10) positive in 51% of all hazelnut positive samples, Ara h 8 (PR-10) in 33% of all peanut positive cases. The results show that all cases positive for PR-10 components from hazelnut or peanut source were also positive for birch extract, suggesting that Birch (r Bet v 1) screening might possibly be introduced in early screening. This is applicable, only in cases where hazelnut or peanut allergy based on clinical history can be excluded. In 2015, there were in total 483 inhalations screenings (Phadiatop) and were positive in 51% of cases. OAS as a symptom was recognized in 1% of all requests. In 69% of cases specificity of the allergy was unknown and in 31% known. In 90% of cases where allergy specificity was known from the clinical history, the ordering physicians were allergy specialists.
Discussion
Additional value of the algorithm
Increase of pre-test probability
The challenge in allergy disease management and unmet clinical need is how to increase the pre-test probability. Although the algorithm developed by our group in 2014 does not provide pre-test probability on an individual patient level, it can increase pre-test probability in general, giving guidance to clinicians and general practitioners. It is not limited to only one or two types of symptoms, but it is more generally applicable, as IgE-mediated allergy disease can present with various symptoms (2). Most of these symptoms are included in the algorithm presented as it embraces six different symptom categories. Some symptoms of IgE disease like conjunctivitis, eosinophilic esophagitis, venom and drug allergy are not classified as symptoms in our approach. The allergens associated with e.g. fish, venom or drug allergy, including their MCs, can be requested but not automatically as reflex testing in this algorithm.
In 2016, a position paper from the EAACI was published underlining the need for approaches to increase pre-test probability of allergic disease, but are not yet developed (2). This position paper states that clinical history is essential for decisions about the most appropriate IgE sensitization tests. These may be skin prick test or serum specific IgE. Patient specific internal factors (e.g., gender, age, presenting features of allergic reaction and co-existing diseases) and external factors (e.g., stress, viral infection) are important. All these factors may influence the relationship between IgE results and probability of clinical allergy (possibly in a non-linear relation). Therefore, it is difficult to derive a pre-test probability at individual patient level. Until now, all nomograms and prognostic models developed for specific settings do not apply for different circumstances and regions (due to different settings: environmental, test population, global position with respect to the equator, rural or not rural setting etc.) (2,12,13).
Contribution to value based health care in allergy
Many different allergen extract mixtures are requested by (inexperienced) specialists/general practitioners without a clear relation between symptoms and cause. When positive, all relevant extracts are tested automatically generating high costs and confusion on how to interpret so many results. Also, inappropriate dietary advices are given, negatively influencing patients’ quality of life. It is to be expected that the testing by means of MCs will further increase, following developments in the field of allergy. By applying this algorithm, laboratory screening costs may at first rise as a result of the increase in the number of MCs tested, as shown in this study (Figure 2). Though, appropriate use of the algorithm may result in an overall healthcare cost reduction, as it can explain cross-reactivity and when interpreted correctly avoid giving incorrect dietary advice and referral to allergy specialists.
Using OAS more frequently as a symptom
An important condition of efficient use and additional value of this algorithm is the recognition of OAS as a symptom. Our results show, that after introducing the algorithm little shift has taken place in the request pattern of specialists (paediatric, dermatology, E.N.T. and allergy), especially with respect to recognition of OAS, even after longer application of the algorithm. More training, explanation and promotion of OAS could help to better recognize the symptoms for general practitioners and other specialists. OAS symptoms should be more widely advocated. For some food allergens, early testing of PR-10 from birch (especially in areas of high prevalence of birch allergy) can be helpful to reduce the number of MCs screened, although co-existing allergies (for example with hazelnut or peanut) cannot be fully excluded based on only serological screening and should be obtained from clinical history.
Considerations and limitations of the algorithm
Evaluation period
Although it might seem that a longer period of comparison (for example six months) instead of only two months period would strengthen our findings/conclusions, no big difference is to be expected with respect to the request pattern or general conclusion. First, in 2014, there was little knowledge about MCs and if they were requested it was upon advice allergy specialist as retrieved from the records. Second, all information about patient symptoms had to be manually retrieved from patient records and categorized. This categorization is not easy because records are not standardized and specialist-dependent. After introduction of the algorithm, in 2015, a standardized approach is used and the types of symptoms are easy to recognize and count. Therefore, we chose two months for comparison, which are considered sufficiently, but with respect to the symptoms we evaluated the whole of 2015. The possibility to choose OAS as a symptom is given, but this option was hardly ever chosen.
Acute eczema/atopic dermatitis inclusion in algorithm
Atopic dermatitis (also known as atopic eczema and as such used in our country) is a highly complex disease with not yet understood immunologic background, similar phenotype but many endotypes (14-16). The function of the epidermal barrier and the immune system play an important role in the contribution of IgE-mediated sensitization. Early and proactive management of both mechanisms could improve outcome and quality of life in atopic patients (15,16). Acute eczema/atopic dermatitis is an aspect in allergic disease upon which recommendations and guidelines (national and international) are not conclusive. Some recommend screening only in children, where others recommend screening for IgE sensitization only to food allergens in relation to atopic dermatitis in children and adults, especially if supported by clinical history and acute course (17-20). This was/is also the general recommendation in the Netherlands and therefore acute eczema/atopic dermatitis was included in this algorithm.
Actuality of advice and refinement of cut-off’s
Automatic generation of reflex testing (like MCs) and commentary texts should be periodically revised. Recommendations are changing rapidly, as for example the recent one supporting peanut exposure at early age in small children (21). Furthermore, a refinement with respect to cut-off’s use for extracts and the use of specific activity can further enrich the quality of obtained information (22).
Cost-effectiveness study
To really measure the effect of the algorithm, the practitioners should be questioned about the applicability of the algorithm as well as a cost-effectiveness and process evaluation study. The benefit of introduction of the algorithm shall theoretically result in less referrals to dieticians and allergists and/or ultimately to less prescriptions of epinephrine injections. Moreover, it might lead to improvement of patients’ quality of life. These aspects are still to be evaluated.
Conclusions
We developed an algorithm based on clinical symptoms alongside information regarding the possible sensitization of the patient, which uses reflex testing in allergy diagnostics and automatically generates an interpretative comment. The algorithm increases the pre-test probability of allergic disease at a general level by combining symptoms and screening results. Therefore, this algorithm can be seen as a diagnostic tool, helpful in the management of atopic patients.
Acknowledgments
The authors thank the members of the Immunochemistry group, Department of Clinical Chemistry, Kees van der Laan, Reinier Bossché and Touria Souafi for their technical assistance during the process of developing and testing the algorithm. We also like to thank Niahm O’Sullivan for her language editing assistance and allergy specialist A. Ferket for her commentary.
Funding: None.
Footnote
Conflicts of Interest: All authors have completed the ICMJE uniform disclosure form (available at http://dx.doi.org/10.21037/jlpm.2017.08.07). The authors have no conflicts of interest to declare.
Ethical Statement: The authors are accountable for all aspects of the work in ensuring that questions related to the accuracy or integrity of any part of the work are appropriately investigated and resolved. The study was conducted in accordance with the Declaration of Helsinki (as revised in 2013). The Executive Committee of the Medical Ethical Committee, has reviewed on February 22nd, 2017 and Board of Directors approved the Research Protocol (Protocol T2017-14).
Open Access Statement: This is an Open Access article distributed in accordance with the Creative Commons Attribution-NonCommercial-NoDerivs 4.0 International License (CC BY-NC-ND 4.0), which permits the non-commercial replication and distribution of the article with the strict proviso that no changes or edits are made and the original work is properly cited (including links to both the formal publication through the relevant DOI and the license). See: https://creativecommons.org/licenses/by-nc-nd/4.0/.
References
- Matricardi PM, Kleine-Tebbe J, Hoffmann HJ, et al. EAACI Molecular Allergology User's Guide. Pediatr Allergy Immunol 2016;27:1-250. [Crossref] [PubMed]
- Roberts G, Ollert M, Aalberse R, et al. A new framework for the interpretation of IgE sensitization tests. Allergy 2016;71:1540-51. [Crossref] [PubMed]
- Bousquet J, Hellings PW, Agache I, et al. ARIA 2016: Care pathways implementing emerging technologies for predictive medicine in rhinitis and asthma across the life cycle. Clin Transl Allergy 2016;6:47. [Crossref] [PubMed]
- Bousquet J, Schunemann HJ, Fonseca J, et al. MACVIA-ARIA Sentinel NetworK for allergic rhinitis (MASK-rhinitis): the new generation guideline implementation. Allergy 2015;70:1372-92. [Crossref] [PubMed]
- Bianchi A, Tsilochristou O, Gabrielli F, et al. The Smartphone: A Novel Diagnostic Tool in Pollen Allergy? J Investig Allergol Clin Immunol 2016;26:204-7. [Crossref] [PubMed]
- Larenas Linnemann DE, Medina Avalos MA, Lozano Saenz J. How an online survey on the treatment of allergic rhinitis and its impact on asthma (ARIA) detected specialty-specific knowledge-gaps. World Allergy Organ J 2015;8:18. [Crossref] [PubMed]
- Muraro A, Dubois AE. EAACI Food Allergy and Anaphylaxis Guidelines. Food allergy health-related quality of life measures. Allergy 2014;69:845-53. [Crossref] [PubMed]
- Van Hoecke H, Vandeplas G, Acke F, et al. Dissemination and implementation of the ARIA guidelines for allergic rhinitis in general practice. Int Arch Allergy Immunol 2014;163:106-13. [Crossref] [PubMed]
- Custovic A, Johnston SL, Pavord I, et al. EAACI position statement on asthma exacerbations and severe asthma. Allergy 2013;68:1520-31. [Crossref] [PubMed]
- Kristiansen M, Dhami S, Netuveli G, et al. Allergen immunotherapy for the prevention of allergy: A systematic review and meta-analysis. Pediatr Allergy Immunol 2017;28:18-29. [Crossref] [PubMed]
- Subbarayal B, Schiller D, Mobs C, et al. Kinetics, cross-reactivity, and specificity of Bet v 1-specific IgG4 antibodies induced by immunotherapy with birch pollen. Allergy 2013;68:1377-86. [Crossref] [PubMed]
- DunnGalvin A, Daly D, Cullinane C, et al. Highly accurate prediction of food challenge outcome using routinely available clinical data. J Allergy Clin Immunol 2011;127:633-9.e1-3.
- Posa D, Perna S, Resch Y, et al. Evolution and predictive value of IgE responses toward a comprehensive panel of house dust mite allergens during the first 2 decades of life. J Allergy Clin Immunol 2017;139:541-9.e8. [Crossref] [PubMed]
- Bieber T, D'Erme AM, Akdis CA, et al. Clinical phenotypes and endophenotypes of atopic dermatitis: Where are we, and where should we go? J Allergy Clin Immunol 2017;139:S58-64. [Crossref] [PubMed]
- Muraro A, Lemanske RF Jr, Hellings PW, et al. Precision medicine in patients with allergic diseases: Airway diseases and atopic dermatitis-PRACTALL document of the European Academy of Allergy and Clinical Immunology and the American Academy of Allergy, Asthma & Immunology. J Allergy Clin Immunol 2016;137:1347-58. [Crossref] [PubMed]
- Weidinger S, Novak N. Atopic dermatitis. Lancet 2016;387:1109-22. [Crossref] [PubMed]
- Bieber T. Why we need a harmonized name for atopic dermatitis/atopic eczema/eczema! Allergy 2016;71:1379-80. [Crossref] [PubMed]
- Chan S, Cornelius V, Chen T, et al. Atopic Dermatitis Anti-IgE Paediatric Trial (ADAPT): the role of anti-IgE in severe paediatric eczema: study protocol for a randomised controlled trial. Trials 2017;18:136. [Crossref] [PubMed]
- Katayama I, Aihara M, Ohya Y, et al. Japanese guidelines for atopic dermatitis 2017. Allergol Int 2017;66:230-47. [Crossref] [PubMed]
- Laske N, Niggemann B. Does the severity of atopic dermatitis correlate with serum IgE levels? Pediatr Allergy Immunol 2004;15:86-8. [Crossref] [PubMed]
- Fleischer DM, Sicherer S, Greenhawt M, et al. Consensus communication on early peanut introduction and the prevention of peanut allergy in high-risk infants. Allergy 2015;70:1193-5. [Crossref] [PubMed]
- Kos S, Sanders RJ, Neele M, et al. Preliminary study in specific activity of molecular components in allergy: implications for diagnostics and relationship with disease severity. Clin Chem Lab Med 2017;55:e113-7. [Crossref] [PubMed]
Cite this article as: Kos S, Neele M, Phaff R, Mertens S, Schenk R, Wulkan R. Allergy algorithm to increase pre-test probability of allergic disease. J Lab Precis Med 2017;2:64.