Red blood cell distribution width (RDW)—a mechanism for normal variation and changes in pathological states
Introduction
The red blood cell distribution width (RDW) is an objective measure of anisocytosis which can change with a number of disease processes involving the formation, in vivo change and removal of red blood cells (RBC) from the circulation. Many papers have matched changes in the RDW with other measured factors using clinical correlations, changes in the enzyme and physical characteristics of the RBC and also complex models linking causes across multiple fields (1,2). Many of the causes of variation in RBC are still unclear. RDW changes occur early in anaemias (3), renal (4), cardiac (5), respiratory (6), hypoxic (7), malignant (8) and other diseases (9) and also with age (10). Where inflammation is a factor, the level of C-reactive protein (CRP) is raised more or less in parallel with the RDW but there are other conditions and normal states where the CRP is normal but the RDW raised (10,11). Presumably all these conditions affect RBC through their lifespan, but there is still no agreed mechanism. Other authors (12) suggest that changes in RBC are part of a homeostatic response to disease, but the RDW also changes in aging without clinical disease (11). The large number of papers seeking an answer and their high quality suggest that we should seek some other explanation. Biological regulation is a well-recognised field (13,14) and Weatherall has suggested (15) RBC are part of this system. Rather than consider the biochemical and physical processes, we have taken a minimalist approach to the basic processes that influence RBC survival. We have taken the three basic items of entry volume, rate of decline in volume and exit volume. We have not sought the factors determining these values, but rather the changes in standard outputs when the levels or the distributions of one or more of these factors change. In addition to the mean cell volume (MCV), RDW and mean RBC lifespan, we have also modelled the changes in the distributions of RBC volumes and lifespans. We have shown that changes in the regulation of RBC coupled with random variation of the input values can explain many known findings.
Methods
The model
The model is a computer program (see Appendix 1) which is available on request. The input data for each individual RBC entering the circulation are the entry volume, the daily loss of volume as a fraction of the current volume and the cell volume that triggers removal from the circulation and also a variance for each of these values. For each RBC, the change in volume is calculated day by day from entry to exit and the daily volume recorded. In most runs, data were collected on 200 RBC. The outputs were the mean values and distributions of the MCV, RDW and lifespan of the RBC.
Results
The model was calibrated using results from normal subjects to obtain reasonable initial estimates for the three input variables. For the initial modelling, we assumed that each factor had an exact value (CV =0; Table 1). The outputs for the mean values of the MCV, RDW and RBC lifespan were acceptable, but the distributions for the RBC volumes and lifespans did not match published results (Figures 1,2).
Table 1
RBC entry volume (fL) | RBC exit volume (fL) | Daily volume loss (%) | CV% | MCV (fL) | RDW% | Mean lifespan (days) |
---|---|---|---|---|---|---|
110 | 73 | 0.37 | 0 | 90.7 | 12.4 | 114 |
110 | 73 | 0.37 | 7 | 90.2 | 12.9 | 114 |
RBC, red blood cell; CV, coefficient of variation; RDW, red blood cell distribution width; MCV, mean cell volume.
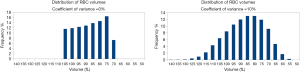
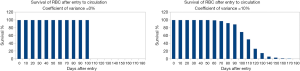
These inputs and mean outputs are all within the normal range. However, with exact values for the input factors, when we plotted the volume distribution and lifespans of the RBC the plots differed widely from accepted patterns. The shape and spread of the curve for cell volumes do not match known distributions. It shows central crowding and lacks the upper and lower tails shown in distributions of real data. As the 200 cells were identical in the model, they all remained in the circulation for the same duration and then were all removed on the same day. Research studies show that normal RBC have a mean life span of about 115 days, but the range is about 70 to 140 days. Although this model gives the correct results for standard measures, it is obviously wrong. The mean values of outputs are correct, but the shapes of the distributions do not match published data. The fault lies in assuming that each cell has an identical value for entry volume, exit volume and daily loss. In biology, most variables have a range of values, rather than a single exact value. To allow for this, we say that a variable has a normal mean value and a range of +/− 2 standard deviations. When we do this for the model, the outcome is very different.
The model did not match the observed and the cause lay in the assumption that the cells were identical. In biology, normal values are seldom exact but have a mean and a range of values. When we applied this to the input values of the model, the results changed (Table 1 and Figures 1,2).
The mean value of each output remained almost the same (Table 1), but the distributions of RBC volumes and lifespan changed. When each input factor had a mean value and a coefficient of variation of 5%, the distributions matched published research.
Modelling pathological conditions and their effects on the RDW
Iron deficiency anaemia
We used known data for changes in RDW with various conditions to test the model. There may be several mechanisms that cause changes in the RDW with different pathologies. The simplest is iron deficiency anaemia. In this condition, the input of normocytic iron-replete cells is gradually replaced by microcytic iron-deficient RBC. The first change in the full blood count (FBC) is an increase in the RDW followed later by a decrease in the MCV mean corpuscular haemoglobin (MCH) and mean corpuscular haemoglobin concentration (MCHC) (3). The circulating population is a mixture of these two cell types. When this was run on the computer model the results were as shown in Table 2. As the proportion of microcytes increases, there is a slow fall in the MCV, almost no change in lifespan, but a large and early increase in RDW. Clinically, the increase in RDW would precede the fall in MCV. Whenever two populations with different means combine, the standard deviation changes more rapidly than the mean.
Table 2
Microcytes, % | MCV (fL), change (%) | RDW%, change (%) | Lifespan (days), change (%) |
---|---|---|---|
0 | 90.2, 0 | 12.4, 0 | 114, 0 |
5 | 89.1, −1 | 13.7, +10 | 114, 0 |
10 | 88.2, −2 | 14.3, +15 | 115, +1 |
20 | 86.1, −5 | 15.8, +27 | 115, +1 |
50 | 80.5, −11 | 17.7, +43 | 119, +4 |
For all percentages of microcytes the input vales were as follows: normocytes entry volume =110 fL, exit volume =73 fL, daily loss =0.37%, microcytes entry volume =90 fL, exit volume =63 fL, daily loss =0.37%. RDW, red blood cell distribution width; MCV, mean cell volume.
Changes in the RDW following a period of hypoxia
A similar mechanism explains the rise in RDW following a pulse of hypoxia which was described by Ycas et al. (7) (Table 3). These authors showed that the hypoxia produced a rise in the erythropoietin level which gave rise to the release of large immature erythrocytes into the circulation. Many of these cells had a shortened lifespan. We modelled the changes following the entry of erythrocytes with an entry volume of 140 fL and a normal lifespan, and the same cells with a shorter lifespan.
Table 3
Cells in circulation | Entry volume (fL) | Daily decrease (%) | Exit volume (fL) | MCV (fL), change (%) | RDW%, change (%) | Lifespan (days), change (%) |
---|---|---|---|---|---|---|
100% normocytes | 110 | 0.0037 | 73 | 90.2, 0 | 12.9, 0 | 114, 0 |
20% large cells, normal daily decrease | 140 | 0.0037 | 73 | 92.2, +2 | 15.3, +19 | 117, +3 |
20% large cells, fast daily decrease | 140 | 0.0100 | 73 | 90.9, +1 | 14.1, +9 | 111, −3 |
The CV was 5% throughout. CV, coefficient of variation.
With the influx of large cells with a normal lifespan, there was a large increase in the RDW and lesser rise in the MCV. With the shorter lifespan there was a smaller but significant rise in the RDW and almost no change in the MCV.
Other disease states and the RDW
The examples above show the effects on the distribution of RBC volumes when two populations with different means are mixed together. However, there is no evidence of this simple statistical mechanism in other disease states where the RDW rises.
Biological regulation is a necessary feature for all organisms. It is what allows an organism to handle the effects of change, altering its own dynamics in response to changes in internal and external conditions. But regulation can never be exact, and biological regulation is even less exact than most man-made systems. In almost all biological fields, the value of variable is defined by its mean and probability distribution. With tight regulation, this range is narrow, but maintaining this needs information and energy. When these are lacking the system becomes “slack” and there are wider limits to the range of “normality”.
Our model has shown that “randomness” or unexplained variation in levels must be present to explain observed data. It also shows that the RDW increases when the standard deviations of the input variables increased but the means of the variables are largely unchanged. We suggest that significant disease leads to some loss of control of these biological processes, which allows great variation about mean values. Table 4 shows this effect.
Table 4
CV% | MCV (fL), change (%) | RDW%, change (%) | Median life span (days), change (%) |
---|---|---|---|
0 | 90.3, 0 | 11.9, 0 | 112, 0 |
5 | 90.2, −0.1 | 12.6, +6 | 114, +2 |
10 | 90.1, −0.2 | 15.4, +29 | 115, +3 |
15 | 90.3, 0 | 18.7, +57 | 117, +4 |
20 | 90.9, +1 | 22.0, +85 | 120, +7 |
The levels of RBC entry volume =110 fL, RBC exit volume =73 fL and daily volume loss =0.37% are unchanged throughout. CV, coefficient of variation; RBC, red blood cell; RDW, red blood cell distribution width; MCV, mean cell volume.
When we increase the coefficient of variation, that is, we decrease the control over the level of all input variables so that they have wider ranges, then the RDW increases by 85% while the MCV remains constant and the mean lifespan increases by 7%. No matter what the cause of the increased range of inputs, the RDW is selectively increased. The increase in the lifespan agrees with the findings of Patel et al. (11).
In the previous table all variables were increased by the same amount. When we keep the same mean level of each of the input variables but increase the range on each input variable separately, we get different results (Table 5).
Table 5
Input volume, CV% | Daily decrease, CV% | Exit volume, CV% | MCV (fL), change (%) | RDW%, change (%) | Lifespan (days), change (%) |
---|---|---|---|---|---|
5 | 5 | 5 | 90.2, 0 | 11.6, 0 | 114, 0 |
20 | 5 | 5 | 94.3, +5 | 18.2, +57 | 108, −5 |
5 | 20 | 5 | 90.2, +0 | 11.6, +0 | 112, −2 |
5 | 5 | 20 | 86.6, −4 | 15.1, +30 | 115, +1 |
The mean levels of inputs were constant RBC entry volume =110 fL, RBC exit volume =73 fL, daily volume loss =0.0037%. CV, coefficient of variation; RDW, red blood cell distribution width; MCV, mean cell volume.
The output levels of MCV, RDW and lifespan changed in different ways when the randomness of individual inputs was altered. The greatest change was when the CV of the input volume was set at 20% and the least change when the CV of the daily volume loss was set at 20%.
Discussion
Many research papers have shown that the level of RDW correlates with the severity and mortality rates of both acute and chronic disease, but no mechanism for this correlation has been found (16). Given the amount and quality of this work, further similar studies are unlikely to succeed, A new approach is needed.
We have developed a computer model which uses only basic data needed to describe the life cycle of RBC in the circulation. We have shown how changes in some of these factors alter commonly measured diagnostic results. Some, like rise in RDW with iron deficiency anaemia, arise from the presence of two different populations, although there may also be changes in the randomness of the RBC measures. However, this does not explain the changes in other disease states. We have developed a computer model which shows that random variations in the level of input factors change the RDW but leave other mean values almost unchanged. But there is little gain in replacing and unknown cause for the variations in RDW with an unknown cause for variations in randomness.
Biological regulation is a necessary feature for all organisms (13). It is what allows an organism to handle the effects of change, altering its own dynamics in response to changes in internal and external conditions. But regulation can never be exact, and biological regulation is even less exact than most man-made systems. In almost all biological fields, the value of variable is defined by its mean and probability distribution. With tight regulation, this range is narrow, but maintaining this needs information and energy. When these are lacking the system becomes “slack” and there are wider limits to the range of “normal” (17,18).
Our model has shown that “randomness” or unexplained variation in levels can explain observed data. It also shows that the RDW increases when the standard deviations of the input variables increase but the means of the variables are largely unchanged. We suggest that significant disease leads to some loss of control of these biological processes, which allows great variation about mean values. Table 4 demonstrates this effect.
There is evidence that randomness is not only necessary but also inevitable (17,19). The model has shown that when we use known values acceptable levels for the input factors as correct and exact values, we can get appropriate outputs for the commonly measured parameters such as RDW and MCV standard summary outputs, but the population distribution of RBCs in the circulation does not match experimental results. In Nature, variations between individuals and species rests on differences between the biochemistry of their constituent cells. Without these variations, there would be no evolution (17). Identical twins have the same genes, but they are seldom truly identical, so at some level their identical genes must lead to differing biochemical functioning and outcomes. Random variation of inputs is a possible explanation. Genes regulate biochemical function and regulation, but to do this they need a basic input of materials and energy to manipulate them. Building complex molecules and structures from simpler materials demands large amounts of energy, organisation and regulation. When muscle protein is built from simpler compounds, only 42% of the energy goes into the final muscle molecules, the other 58% is spent on finding, selecting and combining the raw materials and is eventually lost as heat (19,20,21). RBC are more complex that muscle proteins, so the efficiency is probably less and the need for tight regulation greater. The complexity of the process suggests that decrease in regulation would have a large effect on the input. By contrast, the breakdown of complex structures is a simple process that needs minimal energy (16) so is less influenced by outside sources. External factors such as disease, toxins, or lack of raw materials or lack of energy are therefore more likely to affect RBC formation than their reduction in size and exit from the circulation. It may be no coincidence that the main effect of an episode of hypoxia is on RBC formation (7). Our model also shows that an increase in randomness in RBC formation produces a greater increase in RDW than equivalent increasing randomness on exit volumes or rate of volume loss. In general, it is likely that disease and other physiological stresses decrease levels of regulation, the resulting in an increase in randomness and a consequent increase in the variation of the output, measured as the RDW which is a combination of the three input processes used in our model RDW.
Another finding supports the hypothesis of varying levels of regulation. The normal level of the RDW varies with age (see Table 6) (22-24).The levels differ between different laboratories, but within each series the trend is clear and consistent. The RDW is very high in the early fetus, decreases throughout later fetal life and childhood, remains low during early and middle adult life, then increases again in old age. These changes are consistent with increasing efficiency of biological regulation in early life, then a period of stability followed by a gradual loss of regulation in old age.
Table 6
Age range | Mean, RDW% | Change from previous level (%) |
---|---|---|
Series 1 (22) | ||
<34 weeks gestation | 17.8 | 0.0 |
>34 weeks gestation | 16.7 | −1.1 |
Series 2 (23) | ||
0–6 months | 15.1 | 0.0 |
1–6 months | 13.8 | −1.3 |
6–24 months | 13.5 | −0.3 |
2–6 years | 13.3 | −0.2 |
6–12 years | 13.3 | +0.0 |
Series 3 male & female (24) | ||
<18 years | 12.1 | 0.0 |
18–40 years | 12.2 | +0.1 |
41–55 years | 12.2 | +0.0 |
56–70 years | 12.3 | +0.1 |
71–85 years | 12.6 | +0.3 |
>85 years | 12.8 | +0.2 |
RDW, red blood cell distribution width.
Computer models have an undeserved reputation for producing useful results. Their only role is to generate new hypotheses, then to check these against known data, and, if they pass that test, suggest experiments to verify these new hypotheses with new data. The model should be based on sound scientific principles and knowledge. Predictions that do not match future experimental results show the limitations of the model. If there are many mis-matches then the model is not useful. If the predictions cannot be tested, then the model has little value. Our model can explain some existing data, but to be valid must correctly predict the results of new experiments.
RBC have many features that make them suitable for the study of biochemical regulation. They are complex structures, so their formation is susceptible to dysregulation, they are short lived, so respond rapidly to change, there are readily available methods to get numerical data about their properties and finally there is a vast source of data already and potentially available.
The model
Acknowledgments
Funding: None.
Footnote
Conflicts of Interest: Both authors have completed the ICMJE uniform disclosure form (available at http://dx.doi.org/10.21037/jlpm.2018.08.03). Tony Badrick serves as an unpaid editorial board member of Journal of Laboratory and Precision Medicine from December 2016 to November 2018. The authors have no other conflicts of interest to declare.
Ethical Statement: The authors are accountable for all aspects of the work in ensuring that questions related to the accuracy or integrity of any part of the work are appropriately investigated and resolved.
Open Access Statement: This is an Open Access article distributed in accordance with the Creative Commons Attribution-NonCommercial-NoDerivs 4.0 International License (CC BY-NC-ND 4.0), which permits the non-commercial replication and distribution of the article with the strict proviso that no changes or edits are made and the original work is properly cited (including links to both the formal publication through the relevant DOI and the license). See: https://creativecommons.org/licenses/by-nc-nd/4.0/.
References
- Higgins JM, Mahadevan L. Physiological and pathological population dynamics of circulating human red blood cells. PNAS 2010;107:20587-92. [Crossref] [PubMed]
- Higgins JM. Red blood cell population dynamics. Clin Lab Med 2015;35:43-57. [Crossref] [PubMed]
- Dugdale AE. Predicting iron and folate anaemias from standard blood testing: the mechanism and implications for clinical medicine in developing countries. Theor Biol Med Model 2006;3:34. [Crossref] [PubMed]
- Lippi G, Targher G, Montagnana M, et al. Relationship between red blood cell distribution width and kidney function tests in a large cohort of unselected outpatients. Scand J Clin Lab Invest 2008;68:745-8. [Crossref] [PubMed]
- Wei XB, Liu PC, He PC, et al. Combined efficacy of C-reactive protein and red blood cell distribution width in prognosis of patients with culture-negative infective endocarditis. Oncotarget 2017;8:71173-80. [PubMed]
- Agarwal S. Red cell distribution width, inflammatory markers and cardiorespiratory fitness: results from the National Health and Nutrition Examination Survey. Indian Heart J 2012;64:380-7. [Crossref] [PubMed]
- Yčas JW, Horrow JC, Horrfe BD. Persistent increase in red cell size distribution width after acute diseases: a biomarker of hypoxemia. Clin Chim Acta 2015;448:107-17. [Crossref] [PubMed]
- Koma Y, Arushi A, Maksuoka H, et al. Increased red blood cell distribution width associates with cancer stage and prognosis in patients with lung cancer. PLoS One 2013;8:e80240 [Crossref] [PubMed]
- Salvagno GL, Sanchis-Gomar F, Picanza A, et al. Red blood cell distribution width: A simple parameter with multiple clinical applications. Crit Rev Clin Lab Sci 2015;52:86-105. [Crossref] [PubMed]
- Franco RS. Measurement of Red Cell Lifespan and Aging. Transfus Med Hemother 2012;39:302-7. [Crossref] [PubMed]
- Franco RS. Changes in the properties of normal human red blood cells during in vivo aging. Am J Hematol 2013;88:44-51. [Crossref] [PubMed]
- Patel HH, Patel HR, Higgins JM. Modulation of red cell population dynamics is a fundamental homeostatic response to disease. Am J Hematol 2015;90:422-8. [Crossref] [PubMed]
- Horrobin DF. Principles of biological control. Aylesbury, UK: Medical and Technological Publishing, 1970.
- Westerhoff HV, Winder C, Messiha H, et al. Systems biology: the elements and principles of life. FEBS Lett 2009;583:3882-90. [Crossref] [PubMed]
- Weatherall DJ. Systems biology and red cells. New Eng J Med 2011;364:376. [Crossref] [PubMed]
- Franklin Bunn H. What can we learn from variation of red cell distribution. Am J Hematol 2015;90:369-70. [Crossref] [PubMed]
- Pilpel Y. Noise in biological systems: pros, cons and mechanisms of control. Methods Mol Biol 2011;759:407-25. [Crossref] [PubMed]
- Speck NA. Stress can be a good thing for blood formation. Cell stem Cell 2016;19:279-80. [Crossref] [PubMed]
- Pullar JD, Webster AJ. The energy cost of fat and protein deposition in the rat. Br J Nutr 1977;37:355-63. [Crossref] [PubMed]
- Roux CZ. Use of theoretical efficiencies of protein and fat synthesis to calculate energy requirement for growth in pigs. Brit J Nutrit 2009;101:895-901. [Crossref] [PubMed]
- Waterlow JC, Millward DJ. Energy cost of turnover of protein and other cellular constituents. In: Energy Transformations in Cells and Organisms. Wieser W, Gnaiger E. editors. Stuttgart: Georg Thieme Verlag, 1989:277-82.
- Tonbul A, Tayman C, Catal F, et al. Red cell distribution width (RDW) in the newborn: normative data. J Clin Lab Anal 2011;25:422-5. [Crossref] [PubMed]
- Nathan DG, Oski FA. Hematology of Infancy and Childhood. 2nd edition. Philadelphia: WB Saunders, 1981:1552-74.
- Lippi G, Salvagno GL, Guidi GC. Red blood cell distribution width is significantly associated with aging and gender. Clin Chem Lab Med 2014;52:e197-9. [PubMed]
Cite this article as: Dugdale AE, Badrick T. Red blood cell distribution width (RDW)—a mechanism for normal variation and changes in pathological states. J Lab Precis Med 2018;3:73.