Past, present, and future of laboratory quality control: patient-based real-time quality control or when getting more quality at less cost is not wishful thinking
The past
The history of medical laboratory testing may be traced back to the ancient Egyptians when skilled healers may have used their tongues to check their patient’s urine for a sweet taste as an indication for diabetes. The quality control (QC) of their analysis would lie strictly with their individual experience in correlating degrees of urine sweetness to the symptoms of hyperglycemia; weight loss, excessive urination, and thirst. More recently, advancements in analytical tools made it possible to develop different QC measurements in order to monitor the accuracy of laboratory test results and provide more reliable information for patient diagnosis and management. Those QC tools typically include the use of liquid QC (LQC) materials with known value assignments that are measured during production of patient results in selected time intervals to assess the analytical accuracy of the given method. Those LQC materials may be lyophilized and reconstituted to liquid state prior to analysis or may be acquired in the liquid state ready for use. When values from these materials fall in the predefined acceptability limits, it is assumed that the analytical measuring system is under control and the patient’s results are reliably accurate for the intended use of the assay.
The described above approach is undeniably superior than training your taste buds, but still suffers from limitations.
The present
LQC matrix
In order to keep LQC materials stable, their production cost-efficient, pricing affordable, and combine as many different analytes as needed in one preparation without their interaction between each other, the preparation of nearly all LQC available on the market include some degree of matrix alterations. This occurs by the addition of stabilizers, preservatives, protease inhibitors, etc., which makes them contrived matrixes. These matrixes may not be of human origin (like human serum or plasma), but rather on readily available and less expensive martials of animal origin (like horse or bovine serum, etc.) and may include dilution with other exogenous compounds. Any, or a combination of these factors, may lead to matrix effects and non-commutability of those materials. The lack of commutability indicates that the observed analytical behavior of LQC material analyzed on more than one lot or more than one measurement procedure differs from that of native patient samples. It is not unusual to see differences in observed bias between patient samples and LQC and that the bias may be in opposite directions. Lack of commutability may create a situation when LQC indicates an unacceptable bias that would prompt rejection of patient results when in fact patient results are not affected (false rejection); or when LQC will not demonstrate any bias, but patient results are significantly biased (no true error detection). It has been reported that statistically significant degrees of non-commutability were observed in over 40% of commercially available LQC materials tested (1). Non-commutability was also observed in certified reference materials that are intended to be used for analytical method harmonization and standardization (2). This can lead to errors in claims of traceability to reference materials with false assurance that different methods are harmonized between each other and provide comparable test results (2). As a result, the International Federation of Clinical Chemistry (IFCC) created a Working Group on Commutability that published recommendations on the assessment of commutability in laboratory medicine (3).
Timing of LQC measurements
There is a plethora of published recommendations on optimizing LQC testing in clinical laboratories which include timing and number of samples included between LQC measurements in order to improve error detection and minimize false rejection (4). However, according to the established guidelines from Clinical Laboratory Improvement Amendment (CLIA), it is sufficient to use only two levels of LQC measured during the production run. As a result, it has been reported that most large academic laboratories are still using this approach established back in 1980s (4). It may be conjectured that none of LQC timing algorithm will be able to reliably detect systematic analytical biases due to the intermittent nature of one-at-a-time measurement control and not a continuous real-time process. Systematic bias may occur during patient sample measurement between LQC events and disappear prior to the next LQC event (5). When systematic bias remains undetected, erroneous patient results may escape detection prior to the next LQC event and be reported. It is also possible that LQC procedures will not detect random bias within the analytical run as they are better designed to detect calibration differences between runs, as well as lot-to-lot variations, which will be displayed as “random bias” or imprecision on LQC monitoring charts.
Acceptance criteria and analytical quality goals
Historically, LQC acceptance criteria were based on rules based on the assumption that laboratory test result distributions are following Gaussian (AKA normal) or near-Gaussian rules. Normal probability distribution is presumed to be a cornerstone of statistical methodology. As with any parametric distribution, normal distribution implies an assumption of a central limit theorem and uses its key parameters of mean, standard deviation (SD), and coefficient of variation (CV) that represent variability and uncertainty of its probabilistic analysis. However, normal distribution does have a limitation that it cannot be reliably applied when a CV exceeds 20%. The other parameters of mean and SD, also cannot be applied to skewed distributions, whereby most analyte LQC result distributions are non-Gaussian (6). In the case of non-Gaussian distribution, it is more acceptable to use power transformations (like Box-Cox) and corresponding statistical algorithms for probabilistic analysis (7).
Nevertheless, it is a standard laboratory practice to use normal distribution parameters as acceptance criteria rules for LQC. It has been reported that many laboratories still use a statistically unreliable ±2 SD rule as a QC metric (4). Considering this observation, the use of “Westgard Rules” that were established by Dr. James Westgard in early 1980s was a monumental step forward. Those rules were based on the very extensive empirical analysis of probabilities of error detection and false rejection using real laboratory test results and simulation techniques. Later, Westgard rules were complemented with the use of Six-Sigma approach and were undoubtedly “state of art” in laboratory QC science of the time. The most advanced laboratory LQC software packages are offering “Westgard Adviser” functionality that allows the use of Six-Sigma, user-defined total error allowable (TEa) for calculations of the optimal LQC acceptance rules and provide estimated rates of false rejection and true error detection for the given rule. However, Westgard rules were all based on normal distribution parameters such as mean, SD, CV and there were no attempts to employ any power transformations to account for skewedness of result distributions. One may argue that laboratory test results distribution in the population is different from the distribution of measured LQC materials and the latter is likely to be Gaussian or near-Gaussian, but it has not been proven experimentally. Distribution graphs of some commercial LQC materials from national reference laboratory are presented in Figure 1. It is quite evident that normal distribution parameters cannot be used for a reliable probabilistic analysis and assessment of LQC performance in those examples.
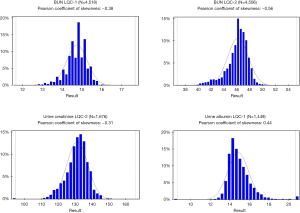
Another issue is related to the choice of proper analytical quality goals like TEa limits. The historical approach has been the use of “one-size-fits-all” quality goals such as 15% or 20% allowance for systematic and/or random bias. This approach is still used in the 2018 FDA Bioanalytical Methods Validation Guidelines for Industry. However, this approach is gradually being replaced by the IFCC recommended multi-model hierarchical approach outlined by a consensus statement from 2015 Strategic Conference of the European Federation of Clinical Chemistry and Laboratory Medicine (8) which emphasize the use of analytical quality goals based on analyte-specific requirements that are established by assessment of different objective models like clinical outcomes, biological variation of analytes, and state of the art. In this approach, a subjective expert opinion model is deemed as least valuable. Unfortunately, many laboratories are still using fixed quality goals criteria for all tests without proper risk and outcome analysis for each analyte (4).
The future
The use of patient’s test results for laboratory QC monitoring was described by early pioneers in this field like Drs. Hoffmann, Waid, and Cembrowski as early as the 1960s (9-12). However, it was rarely implemented in production due to limitations of information technology capabilities, lack of standardization between analytical methods, and low level of motivation among laboratory scientists with a notable exception of hematology testing where Bull’s patient based real-time QC (PBRTQC) algorithm was widely accepted for use in routine QC practices. With the advance of laboratory science, information technology, and improved harmonization/standardization of laboratory methods, the limitations of PBRTQC are now negligible. The common use of “big data” and datamining techniques allowed a shift in laboratory scientist’s mindset to apply patient based QC algorithms and broader acceptance of the need for improvements in laboratory quality monitoring practices. This caused a wave of publications on the use of different variations of PBRTQC techniques and a beginning of new era in the laboratory QC routines. Numerous publications described a variety of flavors for possible PBRTQC optimization approaches including, but not limited to simulated annealing algorithm (13), moving sum of positive patient results (14), and abnormal results distributions (15). Some reference and academic laboratories have begun experimentation with different PBRTQC algorithms and some major laboratory middleware providers started to introduce PBRTQC capabilities in their commercial software packages. This was a start of a change in the entire landscape of laboratory quality practices. One of the major U.S. national reference laboratories in collaboration with one of the major commercial laboratory middleware providers developed and implemented custom PBRTQC protocols in their routine chemistry and immunoassay production practices. Those protocols were successfully assessed for real-time sensitivity (true error detection rate) and specificity (false rejection rate) and later offered by the middleware provider as a commercially available package for the entire IVD market (16).
At first, the PBRTQC implementation process was met with some skepticism among laboratory personnel. This was not unexpected as the entire paradigm of traditional laboratory QC practice was challenged and prior experience with conventional LQC did not fit with new action protocols. It took several months for laboratorians to realize the advantages of this new approach and gain respect for its ability to timely detect systematic errors even in the event when LQC did not detect an error, significantly reduce the number of repeated samples, and most importantly, eliminate erroneous results that were reported. The detailed description of algorithms and PBRTQC rules set up processes were described previously (16), but it may be worth briefly describing the distinctive feature that was used to eliminate erroneous results reporting. This feature was called “release from the back” meaning that when the predefined number of patient results (called a “block”) passes acceptance rules (error threshold limits), the first result in the string of the block is released for reporting; a new result is then added to the block and a new block is again interrogated, if rules passed again, the first result in the second string is released, but if the rules for the given block fails, no result is released and testing is stopped for troubleshooting. This creates a “moving block” of results when only results that pass the acceptance criteria are released and no erroneous results are reported to clients. Figure 2 represents an example of “release from the back” approach.
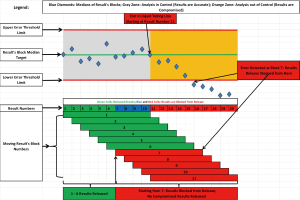
When the PBRTQC protocols performance was assessed after a few years of production, the results were gratifying: the utilization of traditional LQC materials was reduced by approximately 75–85% that translated into significant cost savings, repeat sample analysis was reduced by approximately 50% which translated into significant labor savings (16). Over the period of almost four months post implementation in our laboratory network across the country, a total of 60 delta checks were performed when the PBRTQC rules were violated, affected samples were repeated on a different instrument, and false rejection rates were calculated. Repeated results were compared with results from the failed blocks and a true failure was determined when the observed difference for at least one result was greater than TEa chosen for the given analyte. Only a single delta check out of 60 (1.7%) did not confirm the true failure. The same comparison exercise was performed over the same four-month period when LQC failure resulted in repeating the affected samples on a different instrument. A total of 81 LQC failures with delta checks were performed and 25 (30.9%) were not confirmed as a true failure when the result difference did not exceed the TEa for the given analyte. This indicates that PBRTQC may be superior to LQC in its specificity. It may be more difficult to assess sensitivity of PBRTQC protocols in a production environment. However, during the PBRTQC rules validation studies, the experimental biases were added to patient’s results with error detection rates of 100% with affected results blocked from reporting. The only limitation of this approach is that applied systematic errors were consistent and equal to 1.2 times TEa for the given analyte which may not be the case in a real-time production scenario.
Another important feature of PBRTQC is the ability to connect all instruments in a network and remotely monitor performance of each instrument in real-time, which allows for centralized control of the entire analytical process and timely detection of warning signals such as drifts prior to encountering a process failure. An example of a drift that was detected, the analysis interrupted, and followed by analytical problem resolution can be seen in Figure 3.
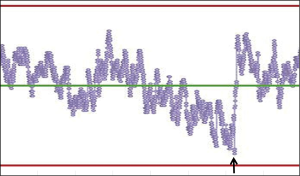
PBRTQC algorithms are also proven to be robust tools in controlling for result shifts due to reagent lot changes and changes in reagent components. During first few years post implementation, standardized chemistry and immunoassay testing was monitored with PBRTQC throughout the laboratory network and observed data was used to significantly improve the manufacturer’s reagent performance and minimize imprecision and bias of results over time. In working with reagent suppliers, the net effect improved the quality of their measurement system.
The described paradigm change in the future of the clinical laboratory QC practices may take time for acceptance by laboratorians, but the long-term results, improve the over-all quality the test result and ultimately improve the benefit to the patient. It was recently reported that statistical literacy among academic pathologists is far from being perfect and many of them possess only basic level of statistical knowledge and will benefit from additional statistical training (17). It is not uncommon that clinicians are confused with the possible root cause of an aberrant test result that does not fit the clinical presentation of the patient or expected treatment outcome. In this case, the burden of explanation is placed on the laboratory to provide an adequate answer. Unfortunately, it is impossible to bring the chance of laboratory error to zero, but when laboratory quality assurance practices are utilizing multiple layers of process control and rely on the modern statistical principles, it is very possible to shrink this chance to a near zero, and PBRTQC is one of the available and effective tools to achieve this goal. Therefore, it is important that laboratories will work with the health care providers to help them better understand the role of PBRTQC in the improvement of healthcare, and provide more accurate results that are truly reliable for their intended clinical use. Another critical point that is often missed in the interaction between laboratories and health care providers is the mutual agreement and understanding on the proper setting of analytical quality goals for the given analyte that will fit the intended clinical use of the test. This dialog can aid in providing better quality healthcare for our patients. Perhaps it is time to change the one-size-fit-all practice for analytical quality goals that so many institutions are still adhering to. It is important to note that IFCC recently created a subcommittee on PBRTQC as a part of its Laboratory Medicine Committee on Analytical Quality that endorsed the use of PBRTQC in clinical laboratories and provided first of its kind recommendations on PBRTQC implementation (18). The new QC era key words are evidence-based, risk-based, and outcome-based.
Acknowledgments
Funding: None.
Footnote
Provenance and Peer Review: This article was commissioned by the Guest Editor (Mark A. Cervinski) for the series “Patient Based Quality Control” published in Journal of Laboratory and Precision Medicine. The article has undergone external peer review.
Conflicts of Interest: Both authors have completed the ICMJE uniform disclosure form (available at http://dx.doi.org/10.21037/jlpm-2019-qc-03). The series “Patient Based Quality Control” was commissioned by the editorial office without any funding or sponsorship. The authors have no other conflicts of interest to declare.
Ethical Statement: The authors are accountable for all aspects of this work in ensuring that questions related to the accuracy or integrity of any part of this work are appropriately investigated and resolved.
Open Access Statement: This is an Open Access article distributed in accordance with the Creative Commons Attribution-NonCommercial-NoDerivs 4.0 International License (CC BY-NC-ND 4.0), which permits the non-commercial replication and distribution of the article with the strict proviso that no changes or edits are made and the original work is properly cited (including links to both the formal publication through the relevant DOI and the license). See: https://creativecommons.org/licenses/by-nc-nd/4.0/.
References
- Miller WG, Erek A, Cunningham TD, et al. Commutability limitations influence quality control results with different reagent lots. Clin Chem 2011;57:76-83. [Crossref] [PubMed]
- Miller WG, Myers GL. Commutability still matters. Clin Chem 2013;59:1291-3. [Crossref] [PubMed]
- Nilsson G, Budd JR, Greenberg N, et al. IFCC Working Group Recommendations for Assessing Commutability Part 2: Using the Difference in Bias between a Reference Material and Clinical Samples. Clin Chem 2018;64:455-64. [Crossref] [PubMed]
- Rosenbaum MW, Flood JG, Melanson SEF, et al. Quality Control Practices for Chemistry and Immunochemistry in a Cohort of 21 Large Academic Medical Centers. Am J Clin Pathol 2018;150:96-104. [Crossref] [PubMed]
- Yago M, Alcover S. Selecting Statistical Procedures for Quality Control Planning Based on Risk Management. Clin Chem 2016;62:959-65. [Crossref] [PubMed]
- Frey CH, Rhodes DS. Quantitative Analysis of Variability and Uncertainty in Environmental Data Models. Volume 1. Theory and Methodology Based Upon Bootstrap Simulation. Report No.: DOE/ER/30250-Vol.1 for U.S. Department of Energy Office of Energy Research. Germantown, MD 20874. April 1999.
- Olivier J, Norberg MM. Positively Skewed Data: Revisiting the Box-Cox Power Transformation. Int J Psychol Res 2010;3:68-75. [Crossref]
- Sandberg S, Fraser CG, Horvath AR, et al. Defining analytical performance specifications: Consensus Statement from the 1st Strategic Conference of the European Federation of Clinical Chemistry and Laboratory Medicine. Clin Chem Lab Med 2015;53:833-5. [Crossref] [PubMed]
- Hoffmann RG, Waid ME, Henry JB. Clinical specimens and reference samples for the quality control of laboratory accuracy. Am J Med Technol 1961;27:309-17. [PubMed]
- Hoffmann RG, Waid ME. The "average of normals" method of quality control. Am J Clin Pathol 1965;43:134-41. [Crossref] [PubMed]
- Cembrowski GS, Carey RN. Laboratory Quality Management. Chicago: AACC Press; 1989.
- Cembrowski GS. Use of Patient Data for Quality Control. Clin Lab Med 1986;6:715-33. [Crossref] [PubMed]
- Ng D, Polito FA, Cervinski MA. Optimization of a Moving Averages Program Using a Simulated Annealing Algorithm: The Goal is to Monitor the Process Not the Patients. Clin Chem 2016;62:1361-71. [Crossref] [PubMed]
- Liu J, Tan CH, Badrick T, et al. Moving sum of number of positive patient result as a quality control tool. Clin Chem Lab Med 2017;55:1709-14. [Crossref] [PubMed]
- Katayev A, Fleming JK. Patient results and laboratory test accuracy. Int J Health Care Qual Assur 2014;27:65-70. [Crossref] [PubMed]
- Fleming JK, Katayev A. Changing the paradigm of laboratory quality control through implementation of real-time test results monitoring: For patients by patients. Clin Biochem 2015;48:508-13. [Crossref] [PubMed]
- Schmidt RL, Chute DJ, Colbert-Getz JM, et al. Statistical Literacy Among Academic Pathologists: A Survey Study to Gauge Knowledge of Frequently Used Statistical Tests Among Trainees and Faculty. Arch Pathol Lab Med 2017;141:279-87. [Crossref] [PubMed]
- Badrick T, Bietenbeck A, Cervinski MA, et al. Patient-Based Real-Time Quality Control: Review and Recommendations. Clin Chem 2019;65:962-71. [Crossref] [PubMed]
Cite this article as: Katayev A, Fleming JK. Past, present, and future of laboratory quality control: patient-based real-time quality control or when getting more quality at less cost is not wishful thinking. J Lab Precis Med 2020;5:28.