Comparison of SARS-CoV-2 anti-spike IgG and anti-nucleoprotein IgG seroprevalence amongst a pre-vaccine cohort of healthcare workers at an academic medical center in Boston, Massachusetts
Introduction
Coronavirus disease 2019 (COVID-19), the disease caused by the novel severe acute respiratory syndrome coronavirus 2 (SARS-CoV-2) causes fever, cough, shortness of breath, and fatigue that can quickly become life threatening (1-3). Due to its transient nature, SARS-CoV-2 RNA testing alone, in the absence of serological testing, is not sufficient to assess population-level viral transmission, pathogen exposure, and the public health burden of the pandemic (4). Accurate measurement of antibodies can help us understand the role antibodies play in overall immunity (5,6). Ongoing studies are investigating the durability of antibodies against SARS-CoV-2 and examining what levels confer protective immunity against severe disease and/or reinfection (6-9).
There has been a rapid increase in serological assay availability in the United States via Emergency Use Authorization (EUA) from the Food and Drug Administration (FDA) (4,10). These assays are designed to detect different immunoglobulin classes (IgM, IgG, IgA) or total antibodies to various epitopes of SARS-CoV-2. Epitopes include the spike (S) and nucleocapsid (N) proteins. Serum anti-N antibodies are more robust in individuals with severe disease but may become undetectable during convalescence before anti-S antibodies (11,12). Most currently approved assays are qualitative in nature and employ a threshold to determine the presence of antibodies. Semi-quantitative assays are also available; however, it remains unclear if the pre-defined threshold for current qualitative and semi-quantitative assays is appropriately set in relation to other assays (4,13). Notably, a positivity threshold of 0.49 signal to cut-off ratio (S/CO) is used in Europe for the Abbott anti-N IgG assay, as opposed to the 1.4 S/CO suggested for use in the United States. The World Health Organization (WHO) has released a reference panel for the comparison of several available assays, a necessary step towards standardizing the evaluation of serological data going forward (14).
As instances of reinfection with COVID-19 become more prevalent, accurate measures of seroconversion and concentrations of serum antibodies are of increasing importance (15). We previously assessed the seroprevalence of SARS-CoV-2 anti-N IgG among health care workers (HCWs) at an academic medical center in Boston, Massachusetts, USA using the Abbott SARS-CoV-2 anti-N IgG qualitative assay (8). Abbott Laboratories has been issued EUA for the AdviseDx anti-spike semi-quantitative SARS-CoV-2 IgG II (anti-S IgG) and IgM (anti-S IgM) assays (6). In this study, we aim to evaluate seroprevalence in this population of HCWs using the semi-quantitative anti-S IgG II assay and compare it to the previously assessed quantitative anti-N IgG seroprevalence. We present the following article in accordance with the STROBE reporting checklist (available at https://jlpm.amegroups.com/article/view/10.21037/jlpm-22-19/rc).
Methods
Study design & participants
Serum samples were obtained from an ongoing longitudinal cohort assessing COVID-19 serological status among HCWs at Boston Medical Center (BMC). In brief, participants were adult BMC employees who worked on campus during the first COVID-19 surge in Boston, MA, USA (March 13th–May 31st, 2020). Baseline serum samples were obtained pre-vaccination, between July 13th–26th, 2020, (n=1,743) and have been previously analyzed for the qualitative detection of SARS-CoV-2 anti-N IgG antibodies using the Abbott SARS-CoV-2 IgG chemiluminescent microparticle immunoassay (CMIA). Study recruitment and assay methodology have been described elsewhere (8). Aliquots of 500 µL were bio-banked from the original study and frozen at −80 ℃. For the present study, an aliquot of 500 µL was used for anti-S IgG & IgM analyses.
Following informed consent, participants completed extensive questionnaire data on demographics (age, gender, race, ethnicity) and overall health and COVID-19 related information including comorbidities, symptoms, and reverse transcription-polymerase chain reaction (RT-PCR) testing history between January 1st and May 31st, 2020. Self-reported prior RT-PCR COVID-19 test results completed during the same period were subsequently confirmed, where possible, using electronic medical records. This project was approved by the Institutional Review Board at Boston University Medical Center (BUMC IRB approval number: H-40503). This study was conducted in accordance with the Declaration of Helsinki (as revised in 2013).
For the present investigation, we analyzed the bio-banked baseline serum samples from the 1,743 HCWs for the semi-quantitative detection of SARS-CoV-2 anti-S IgG and the qualitative detection of anti-S IgM. These assays were performed by the clinical pathology laboratory at BMC on the Abbott Architect i2000SR Instrument (Abbott Laboratories, Abbott Park, IL, USA). Assays were run per the manufacturer’s instructions. For both assays, one 500 µL aliquot of serum was thawed at 4 ℃ for 24 hours or room temperature (~20 ℃) for two hours prior to analyses. Each aliquot ultimately went through one freeze-thaw cycle.
Anti-N IgG assay (qualitative)
Previous investigation of serum antibodies within this cohort has been published (8). In brief, serum was tested using the Abbott SARS-CoV-2 IgG (anti-N IgG) assay, a chemiluminescent microparticle immunoassay (CMIA) for the qualitative detection of IgG antibody against the SARS-CoV-2 nucleoprotein. Samples were considered positive with an index value of ≥1.4 (negative <1.4). This assay and corresponding results are referred to as anti-N IgG in this manuscript.
Anti-S IgM assay (qualitative)
The Abbott AdviseDx SARS-CoV-2 IgM (anti-S IgM) assay is a CMIA for the qualitative detection of IgM antibody in human serum against the SARS-CoV-2 spike protein. In this automated assay, participant serum, paramagnetic particles coated with SARS-CoV-2 antigen, and an assay diluent are incubated together during which the antibodies present in the serum sample bind to the antigen. The resulting luminescence is read and resulted as a relative light unit (RLU). Interpretation of positivity is determined by an index value above a predefined threshold (8,9). Anti-S IgM samples were interpreted as positive (index value ≥1.00) or negative (index value <1.00). This assay and corresponding results are referred to as anti-S IgM.
Anti-S IgG II assay (semi-quantitative)
The Abbott AdviseDx SARS-CoV-2 IgG II (anti-S IgG II) assay is a semi-quantitative assay that detects IgG antibodies to the receptor binding domain (RBD) of the S1 subunit on the spike protein of SARS-CoV-2 in human serum using a two-step indirect CMIA. The assay utilizes magnetic microparticles coated with RBD recombinant protein. The mixture is incubated and then washed prior to the addition of an anti-human-IgG antibody conjugated to acridinium. The recorder molecule is incubated prior to a second wash and followed by addition of a triggering solution that generates luminescence. The light is captured and counted to give an RLU. The RLU’s are read off a six-point calibration curve stored on the instrument generating an AU/mL (arbitrary units/milliliter) value. The assay is standardized to a monoclonal antibody concentration (16). Samples were interpreted as positive when ≥50.0 AU/mL or negative when <50.0 AU/mL. This assay is referred to as the anti-S IgG II assay, and results as anti-S IgG.
Precision studies: anti-S IgM & anti-S IgG II
Precision studies were performed on quality control (QC) material supplied by Abbott Diagnostics. The anti-S IgM assay has two levels of QC: negative and positive. Anti-S IgG II has three levels of QC: negative, low positive, and high positive. Intra-day precision was assessed by analyzing 20 replicates of each level of QC on the same day. Inter-day precision was assessed by analyzing QC for 20 days.
Linearity studies: anti-S IgG II
The analytical measuring range (AMR) of the semi-quantitative anti-S IgG II assay is 22.0–25,000 AU/mL. The suggested manufacturer dilution is 1:2, extending the measuring upper limit to 50,000 AU/mL. Dilution studies were carried out using two participants that had elevated serum antibody concentrations. Although the EUA-approved upper limit is 25,000 AU/mL, reagent was received prior to EUA approval and was reported to be 50,000 AU/mL at the time of these analyses. These samples were diluted serially with negative control to seven concentrations. We ran three separate dilution series for each of the elevated samples on the Abbot Architect i2000SR instrument and present a representative series.
Statistical analysis
Questionnaire data were collected and managed in REDCap electronic data capture tools hosted at Boston University, CTSI 1UL1TR001430 (17,18). Imprecision was assessed by measuring mean, standard deviation (SD), and coefficient of variation (CV) for inter and intra-day precision. Linearity data were analyzed using GraphPad Prism software version 9.0 (GRAPH PAD software Inc., California, USA). Quantitative anti-S IgG data were log2-transformed for ease of visualization.
We tested the association between anti-S IgG and sex, race, and gender using a Fisher’s Exact Test and Chi-square analyses. Missing data in this study ranged from 0.12% to 1.49%. Individuals missing responses to these categorical variables and were excluded from analyses of association.
Positive and negative concordance of the anti-S IgG II assay was calculated using molecular testing (RT-PCR) as the gold standard. Comparison between assays was assessed by McNemar’s Chi-squared test. A receiver operating characteristics (ROC) curve was used to assess assay thresholds and define trade-offs in assay sensitivity and specificity using RT-PCR as the gold standard. A P value of <0.05 was considered statistically significant, and all tests were two-sided. Analyses were performed in R Version R-3.5.3 (R Foundation for Statistical Computing). Select figures were produced by GraphPad Prism version (9.0.2) by GraphPad Software (San Diego, USA).
Results
Study population
Study recruitment techniques, participant demographics, and self-reported COVID-19 symptoms have been previously reported (8). In brief, 1,743 BMC HCWs provided serum samples for analysis. A majority were female (74.93%), of normal weight (48.42%), and non-smokers (95.87%). Participants mostly self-identified as White (74.53%), Asian (9.18%) or Black (8.09%) and most were not Hispanic or LatinX (91.45%) (Table 1).
Table 1
Variable | Total, n (column %) | Anti-S IgG II status | P value (all data) | RR (95% CI) (all data)a | |
---|---|---|---|---|---|
Positive, n (row %) | Negative, n (row %) | ||||
Total | 1,743 (100.00) | 126 (7.23) | 1,617 (92.77) | ||
Sex | 0.31 | ||||
Female | 1,306 (74.93) | 102 (7.81) | 1,204 (92.19) | Referent | |
Male | 431 (24.73) | 24 (5.57) | 407 (94.43) | 0.71 (0.46, 1.10) | |
Nonbinary/3rd gender | 3 (0.17) | 0 (0.00) | 3 (100.00) | 0.00 (N/A)** | |
Average age (years) (SD) | 41.16 (12.39) | 41.00 (12.21) | 41.17 (12.41) | 0.53 | |
BMI | <0.001* | ||||
Underweight (<18.5 kg/m²) | 34 (1.95) | 0 (0.00) | 34 (100.00) | 0.00 (N/A)** | |
Normal (18.5–24.9 kg/m²) | 844 (48.42) | 53 (6.28) | 791 (93.72) | Referent | |
Overweight (25.0–29.9 kg/m²) | 528 (30.29) | 29 (5.49) | 499 (94.51) | 0.87 (0.56, 1.36) | |
Obese (>29.9 kg/m²) | 335 (19.22) | 43 (12.84) | 292 (87.16) | 2.04 (1.40, 2.99) | |
Hispanic/LatinX | 0.1 | ||||
Yes | 143 (8.20) | 15 (10.49) | 128 (89.51) | Referent | |
No | 1,594 (91.45) | 109 (6.84) | 1,485 (93.16) | 0.65 (0.39, 1.09) | |
Race | 0.52 | ||||
White | 1,299 (74.53) | 87 (6.70) | 1,212 (93.30) | Referent | |
Asian | 160 (9.18) | 10 (6.25) | 150 (93.75) | 0.93 (0.50, 1.76) | |
Black | 141 (8.09) | 13 (9.22) | 128 (90.78) | 1.38 (0.79, 2.40) | |
Native American/Pacific Islander | 7 (0.40) | 0 (0.00) | 7 (100.00) | 0.00 (N/A)** | |
Other | 123 (7.06) | 12 (9.76) | 111 (90.24) | 1.46 (0.82, 2.59) | |
Smoking | 0.77 | ||||
No | 1,671 (95.87) | 122 (7.30) | 1,549 (92.70) | Referent | |
Yes | 46 (2.64) | 4 (8.70) | 42 (91.30) | 1.19 (0.46, 3.09) |
Column percentages may not always add up to 100% due to missing data of <2.0%. *, statistically significant when P value <0.05; **, N/A: unable to divide by 0. a, relative risk of anti-S IgG positivity. IgG, immunoglobulin G; SD, standard deviation; BMI, body mass index.
Anti-S IgM and anti-S IgG II assay precision
Anti-S IgM negative and positive control exhibited an intra-day CV of 16.5%, and 2.2%, respectively. Anti-S IgM negative and positive control exhibited an inter-day CV of 21.3%, and 1.9%, respectively. Anti-S IgG II negative, low positive, and high positive control exhibited an intra-day CV of 23.9%, 3.3%, and 2.9%, respectively. Anti-S IgG negative, low positive, and high positive control exhibited an inter-day CV of 16.6%, 2.4%, and 2.4%, respectively (Table S1).
Anti-S IgG II assay linearity
We assessed assay linearity of this semi-quantitative assay using two participants’ serum. One participant result was greater than the AMR (46,820 AU/mL) and shows an acceptable linear response with a r-squared value of 0.99 (Figure S1A). Similarly, the second participant result with an original anti-S IgG level of 11,711.60 AU/mL showed acceptable linearity with a r-squared value of 0.99, though this participant did not exceed the AMR (Figure S1B). These data suggest that the differences between expected and measured results are within the 95% confidence interval (CI). Significant differences were observed between expected and observed results below the positivity threshold, specifically at the lower end of the AMR (Figure S2).
Anti-S IgG seroprevalence
Overall, 126 of 1,743 (7.23%) participants were anti-S IgG positive. Participants who were female, Hispanic, or Black were more likely to be seropositive, but these findings did not reach statistical significance. Obese participants were two times more likely to be seropositive for anti-S IgG compared to normal weight participants [RR: 2.04 (95% CI: 1.40–2.99)]. Among all participants, anti-S IgG II results were not associated with age, race, or gender (Table 1, Figure S3).
Anti-S IgG seroprevalence compared to RT-PCR results
A total of 441 participants reported a previous RT-PCR test. Of the 85 RT-PCR positive, 75 were positive for anti-S IgG, corresponding to a positive concordance of 88.2% (95% CI: 79.4–94.2%). Of the 350 RT-PCR negative, 341 were seronegative for anti-S IgG corresponding to a negative concordance of 97.4% (95% CI: 95.2–98.8%) (Table 2, Figure 1). Of the remaining six with indeterminate results, two were positive for anti-S IgG (Table 2). Among participants with no prior RT-PCR (n=1,302), 40 (3.1%) were positive for anti-S IgG antibodies. Independent of demographics, there was a statistically significant difference among the anti-S IgG levels by RT-PCR test result (P<0.001) (Figure 1). It is apparent that participants with a negative RT-PCR test had detectable anti-S IgG antibodies and the relative semi-quantitative distribution of anti-S IgG was variable across all groups of RT-PCR status.
Table 2
SARS-CoV-2 anti-S IgG II result | SARS-CoV-2 RT-PCR result | |||
---|---|---|---|---|
Positive | Negative | INDa | Not tested | |
Positive | 75 | 9 | 2 | 40 |
Negative | 20 | 341 | 4 | 1,262 |
a, indeterminate result. IgG, immunoglobulin G; RT-PCR, reverse transcription-polymerase chain reaction; SARS-CoV-2, severe acute respiratory syndrome coronavirus 2.
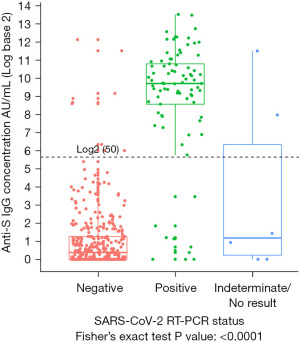
Anti-S IgM seroprevalence compared to RT-PCR results
Of the 85 RT-PCR positive, 47 were also seropositive for anti-S IgM corresponding to a positive concordance of 55.3% (95% CI: 44.1–66.1%). Of the 350 RT-PCR negative participants, 343 were seronegative for anti-S IgM corresponding to a negative concordance of 98.0% (95% CI: 95.9–99.2%). Of the six with indeterminate RT-PCR results, two had detectable anti-S IgM antibodies. Of the 1,302 with no reported RT-PCR test, 20 (1.5%) were positive for anti-S IgM (Table S2).
Days between RT-PCR positive result and serology
Of the 441 individuals with a self-reported prior RT-PCR test, 270 also reported a date of testing. Given the survey collection in May 2020 and serum collection in July 2020, no participants had a reported RT-PCR test dates within 60 days of serology. There was no significant difference between anti-S IgG levels in individuals less than 120 days after positive RT-PCR test result (P=0.16). Anti-S IgG antibodies in RT-PCR confirmed individuals remained elevated over 120 days post infection (Figure S4).
Agreement between anti-N IgG and anti-S IgG status
Overall agreement between the two IgG assays in this population was found to be 97.5% (95% CI: 96.7–98.2%). Of note, the positive agreement was 70.6% (95% CI: 61.8–78.4%), whereas the negative agreement was 99.6% (95% CI: 99.2–99.9%). These two tests were found to be statistically different (test statistic, 21.63, P<0.0001) (Table 3). A total of 37 participants had detectable anti-S IgG antibodies but were negative for anti-N IgG. Of these, 11 participants had a self-reported positive RT-PCR, three had a negative RT-PCR, one had an indeterminate RT-PCR, and 22 were not tested (Table 3).
Table 3
Anti-N IgG results by S/CO ratio | Anti-N IgG result | Anti-S IgG II results | Test statistica | P value | |
---|---|---|---|---|---|
Positive | Negative | ||||
≥1.4 (recommended) | Positive | 89 | 6 | 21.63 | <0.0001 |
Negative | 37 | 1,611 | |||
≥0.49 (adjusted) | Positive | 109 | 21 | 0.32 | 0.5702 |
Negative | 17 | 1,596 |
a, McNemar’s Test Statistic with Yate’s Continuity Correction. IgG, immunoglobulin G; S/CO, signal to cut-off ratio.
Assay threshold optimization
ROC curve analysis showed that the anti-N IgG qualitative assay had an optimal sensitivity and specificity target that were different from the manufacturer specifications. Our data suggest that adjusting the threshold to 0.49 S/CO would result in a sensitivity of 84.7% and specificity of at least 96.9% (Figure S5). Additionally, this threshold change increased the positive agreement between anti-N IgG & anti-S IgG II from 70.6% to 86.5% (95% CI: 79.3–91.9%). It did not, however, appreciably affect the negative agreement which changed from 99.6% to 98.7% (95% CI: 98.0–99.2%). Upon threshold modification, there was no significant difference between anti-N IgG and anti-S IgG II assay results (test statistic, 0.32, P=0.5702) (Table 3, Table S3).
Discussion
This study evaluated the seroprevalence of SARS-CoV-2 anti-S IgG in HCWs using a semi-quantitative assay and compared these findings to previously measured anti-N IgG seroprevalence in the same population. We report an overall anti-S IgG seroprevalence of 7.2% compared to 5.5% for anti-N IgG. Our results demonstrate that the anti-N IgG and anti-S IgG II assays were statistically different. However, lowering the anti-N IgG assay positivity threshold improved overall agreement between the two assays. Taken together, these results support the clinical utility of the anti-S IgG II assay and highlight the potential presence of COVID-19 infection uncaptured by RT-PCR or anti-N IgG assays.
The anti-S IgG II assay shows acceptable intra- and inter-day precision. The negative control precision is expected to be higher, as lower numeric values and small variations resulted in an artificially increased CV. Our results show acceptable linearity up to 50,000 AU/mL which exceeds the upper limit claimed in the package insert. It is currently unclear what titers confer SARS-CoV-2 immunity, but our data suggest that such elevated levels are not rare among HCWs after infection with COVID-19.
Our results indicate less than ideal positive concordance for anti-S IgG II compared with RT-PCR testing [88.2% (95% CI: 79.4–94.2%)]. Further investigation of the discordant samples (n=10) revealed that these participants did not have detectable anti-N IgG, or anti-S IgM antibody. These findings can possibly be explained by mild symptoms and/or disease or early seroconversion. Participants were generally healthy and were not taking medications known to attenuate antibody responses. Literature suggests that the intensity and longevity of antibody response is associated with disease severity (19-21). Of note, survey data of RT-PCR testing history was collected through May 31, 2020, approximately 45 days before serum collection took place beginning July 13, 2020. This discrepancy may affect the classification of participants by RT-PCR status prior to serology. The negative concordance of the anti-S IgG II assay with RT-PCR results was robust.
Among participants with no self-reported RT-PCR test, 40 participants had detectable anti-S IgG antibodies and 23 participants had detectable anti-N IgG antibodies, suggesting a higher sensitivity of the anti-S IgG II assay for identifying past infection which has been corroborated by others (22). Alternatively, this discrepancy between anti-S IgG and anti-N IgG may also be attributable to the shorter half-life of serum anti-N IgG antibodies (7). Ultimately, RT-PCR testing is limited by the brief infectious window during which viral detection is possible. Detectable viral loads during infection via RT-PCR can vary by several factors such as patient medical history, immune response, and medications, whereas antibody measurements can detect exposure to virus for longer periods of time (23-25). Our data support that HCWs in this study were anti-S IgG-positive for at least 120 days after RT-PCR tests.
Neither the anti-N IgG or anti-S IgG II assay results were associated with sex, race, or smoking status. However, the seroprevalence determined by the anti-S IgG II assay was higher (7.2%) relative to the anti-N IgG assay (5.5%) (8). This higher anti-S IgG seroprevalence could also be explained by differences in long-term kinetics between antibodies to different epitopes of the SARS-CoV-2 virus (7). The observed seroprevalence at another Boston area hospital was comparable to ours at approximately 5% from April to June 2020 (23). Whereas others in the Greater Boston region have reported a seroprevalence range between 14% to 25% in June 2020 (26).
Our seroprevalence findings of 7.2% for anti-S IgG and 5.5% for anti-N IgG are markedly higher than other population level IgG seroprevalence findings during similar time frames. In Indiana, one seroprevalence survey estimated 2.79% of the general population was currently or previously infected at the end of April 2020 (27). Additional population-level seroprevalence estimates from June to September 2020 estimate a range from 0.0% in South Dakota to 23.3% in New York had detectable antibodies. Specifically in Massachusetts, this study estimated population seroprevalence at 4.2% (28). Taken together, these findings highlight the increased seroprevalence in our cohort of HCWs, potentially attributable to an increased risk for COVID-19 infection among HCWs, especially at a safety net hospital during the first wave of the pandemic.
The discordance between the anti-N IgG and anti-S IgG II assays prompted us to optimize the pre-set threshold. Upon changing the anti-N IgG threshold to that which is employed in Europe, this assay exhibited better agreement with anti-S IgG II assay. A study conducted by Public Health England independently suggested the same optimized threshold to increase the sensitivity of the assay (29). It is known that antibodies to the SARS-CoV-2 nucleoprotein are a robust marker for acute infection but may wane quicker than anti-S antibodies, specifically anti-S1 RBD IgG antibodies (11). Additionally, these anti-S antibodies are shown to be correlated with neutralization (30,31). These understandings coupled with our findings lend further support for optimizing the assay threshold to achieve better performance characteristics.
These differences between serological assays are also observed between vendors. Comparisons of semi-quantitative SARS-CoV-2 IgG assays have found that the results are not interchangeable despite good correlation to neutralizing antibodies for some (9,10,32,33). Collectively, this highlights the need for harmonization between all SARS-CoV-2 serological assays. Adhering to the WHO guidelines for standardization between SARS-CoV-2 serological assays will be a crucial step, as this standardization can enable us to draw meaningful conclusions about the role of humoral immunity in assessing the overall pandemic response.
The present study benefited from a large sample size and comprehensive survey data collection. Certain limitations are acknowledged; the gold standard for determining protective antibody status is a virus neutralization test which we were unable to employ as we did not have access to live or pseudo viruses. The study is limited by a cross sectional study design which may under- or overestimate the seroprevalence. We were unable to correlate viral load with quantitative antibody levels which may provide insight into antibody kinetics (34). Additionally, the window between survey data collection and serology allows for the potential onset of un-captured COVID-19 infection. The samples were obtained from a single timepoint preventing characterization of antibody kinetics on performance characteristics; however, a subset of participants is being prospectively followed in three-month intervals which will enable robust future analysis. Lastly, the findings are not generalizable to the larger community and are limited to healthcare providers.
In conclusion, the Abbott SARS-CoV-2 anti-S semi-quantitative IgG assay demonstrated acceptable performance characteristics. The study highlights the possible presence of infection among participants with no RT-PCR testing and those with a negative RT-PCR test. Further, our results hint that there are differences in antibody kinetics by epitope and highlight the need for optimizing thresholds of the qualitative SARS-CoV-2 IgG assay for better agreement between assays by the same vendor. Serological testing such as this can assist in providing a better assessment of the public health burden of COVID-19.
Acknowledgments
We would like to acknowledge the clinical chemistry, phlebotomy, and central receiving staff in the Department of Laboratory Medicine and Pathology at Boston Medical Center for working with the research team to accomplish this study. Abbott Diagnostics provided the reagents for the SARS-CoV-2 IgG II assays.
Funding: The study was funded, in part, by BMC Development Philanthropy Funds for COVID-19 research.
Footnote
Reporting Checklist: The authors have completed the STROBE reporting checklist. Available at https://jlpm.amegroups.com/article/view/10.21037/jlpm-22-19/rc
Data Sharing Statement: Available at https://jlpm.amegroups.com/article/view/10.21037/jlpm-22-19/dss
Conflicts of Interest: All authors have completed the ICMJE uniform disclosure form (available at https://jlpm.
amegroups.com/article/view/10.21037/jlpm-22-19/coif). All authors report that Abbott Diagnostics provided the reagents for the SARS-CoV-2 IgG II assays. SHG and LY report employments by Abbott Laboratories. TCB reports funding support from National Institutes of Health (NIAID award K23AI152930-01A1); grants from Gilead Sciences, Inc. (Protocol GS-US-540-5774) and Fujifilm Pharmaceuticals U.S.A., Inc (Protocol FAVI-COV-US201) which are related in that they are clinical trials of SARS-CoV-2 therapeutics, but do not represent conflicts of interest with this manuscript. CA reports that he is a BMC employee, Chair of the department and President of New England Society of Pathologists. The project was funded by BMC institutional award, funded from the philanthropy office. YK reports BMC internal grant funding funded this work. The authors have no other conflicts of interest to declare.
Ethical Statement: The authors are accountable for all aspects of the work in ensuring that questions related to the accuracy or integrity of any part of the work are appropriately investigated and resolved. This project was approved by the Institutional Review Board at Boston University Medical Center (BUMC IRB approval number: H-40503) and informed consent was taken from all individual participants. This study was conducted in accordance with the Declaration of Helsinki (as revised in 2013).
Open Access Statement: This is an Open Access article distributed in accordance with the Creative Commons Attribution-NonCommercial-NoDerivs 4.0 International License (CC BY-NC-ND 4.0), which permits the non-commercial replication and distribution of the article with the strict proviso that no changes or edits are made and the original work is properly cited (including links to both the formal publication through the relevant DOI and the license). See: https://creativecommons.org/licenses/by-nc-nd/4.0/.
References
- Symptoms 2020. Available online: https://www.cdc.gov/coronavirus/2019-ncov/symptoms-testing/symptoms.html
- Wiersinga WJ, Rhodes A, Cheng AC, et al. Pathophysiology, Transmission, Diagnosis, and Treatment of Coronavirus Disease 2019 (COVID-19): A Review. JAMA 2020;324:782-93. [Crossref] [PubMed]
- Asselah T, Durantel D, Pasmant E, et al. COVID-19: Discovery, diagnostics and drug development. J Hepatol 2021;74:168-84. [Crossref] [PubMed]
- National SARS-CoV-2 Serology Assay Evaluation Group. Performance characteristics of five immunoassays for SARS-CoV-2: a head-to-head benchmark comparison. Lancet Infect Dis 2020;20:1390-400. [Crossref]
- Coste AT, Jaton K, Papadimitriou-Olivgeris M, et al. Comparison of SARS-CoV-2 serological tests with different antigen targets. J Clin Virol 2021;134:104690. [Crossref] [PubMed]
- Perkmann T, Perkmann-Nagele N, Koller T, et al. Anti-Spike Protein Assays to Determine SARS-CoV-2 Antibody Levels: a Head-to-Head Comparison of Five Quantitative Assays. Microbiol Spectr 2021;9:e0024721. [Crossref] [PubMed]
- Van Elslande J, Gruwier L, Godderis L, et al. Estimated Half-Life of SARS-CoV-2 Anti-Spike Antibodies More Than Double the Half-Life of Anti-nucleocapsid Antibodies in Healthcare Workers. Clin Infect Dis 2021;73:2366-8. [Crossref] [PubMed]
- Kataria Y, Cole M, Duffy E, et al. Seroprevalence of SARS-CoV-2 IgG antibodies and risk factors in health care workers at an academic medical center in Boston, Massachusetts. Sci Rep 2021;11:9694. [Crossref] [PubMed]
- Bradley BT, Bryan A, Fink SL, et al. Anti-SARS-CoV-2 Antibody Levels Measured by the AdviseDx SARS-CoV-2 Assay Are Concordant with Previously Available Serologic Assays but Are Not Fully Predictive of Sterilizing Immunity. J Clin Microbiol 2021;59:e0098921. [Crossref] [PubMed]
- Brochot E, Demey B, Touzé A, et al. Anti-spike, Anti-nucleocapsid and Neutralizing Antibodies in SARS-CoV-2 Inpatients and Asymptomatic Individuals. Front Microbiol 2020;11:584251. [Crossref] [PubMed]
- Fenwick C, Croxatto A, Coste AT, et al. Changes in SARS-CoV-2 Spike versus Nucleoprotein Antibody Responses Impact the Estimates of Infections in Population-Based Seroprevalence Studies. J Virol 2021;95:e01828-20. [Crossref] [PubMed]
- EUA Authorized Serology Test Performance: Food and Drug Administration; 2021 [Available online: https://www.fda.gov/medical-devices/coronavirus-disease-2019-covid-19-emergency-use-authorizations-medical-devices/eua-authorized-serology-test-performance
- Eyre DW, Lumley SF, O'Donnell D, et al. Stringent thresholds in SARS-CoV-2 IgG assays lead to under-detection of mild infections. BMC Infect Dis 2021;21:187. [Crossref] [PubMed]
- Establishment of the WHO International Standard and Reference Panel for anti-SARS-CoV-2 antibody. 2020. WHO/BS.2020.2403.
- Hoffmann M, Krüger N, Schulz S, et al. The Omicron variant is highly resistant against antibody-mediated neutralization: Implications for control of the COVID-19 pandemic. Cell 2022;185:447-456.e11. [Crossref] [PubMed]
- Laboratories A. AdviseDx SARS-CoV-2 IgG II 2021. Available online: https://www.fda.gov/media/146371/download
- Harris PA, Taylor R, Thielke R, et al. Research electronic data capture (REDCap)--a metadata-driven methodology and workflow process for providing translational research informatics support. J Biomed Inform 2009;42:377-81. [Crossref] [PubMed]
- Harris PA, Taylor R, Minor BLThe REDCap consortium, et al. Building an international community of software platform partners. J Biomed Inform 2019;95:103208. [Crossref] [PubMed]
- Wang Y, Zhang L, Sang L, et al. Kinetics of viral load and antibody response in relation to COVID-19 severity. J Clin Invest 2020;130:5235-44. [Crossref] [PubMed]
- Ravichandran S, Lee Y, Grubbs G, et al. Longitudinal antibody repertoire in "mild" versus "severe" COVID-19 patients reveals immune markers associated with disease severity and resolution. Sci Adv 2021;7:eabf2467. [Crossref] [PubMed]
- Ibarrondo FJ, Fulcher JA, Goodman-Meza D, et al. Rapid Decay of Anti-SARS-CoV-2 Antibodies in Persons with Mild Covid-19. N Engl J Med 2020;383:1085-7. [Crossref] [PubMed]
- Staines HM, Kirwan DE, Clark DJ, et al. IgG Seroconversion and Pathophysiology in Severe Acute Respiratory Syndrome Coronavirus 2 Infection. Emerg Infect Dis 2021;27:85-91. [Crossref] [PubMed]
- Tahamtan A, Ardebili A. Real-time RT-PCR in COVID-19 detection: issues affecting the results. Expert Rev Mol Diagn 2020;20:453-4. [Crossref] [PubMed]
- Kim JY, Ko JH, Kim Y, et al. Viral Load Kinetics of SARS-CoV-2 Infection in First Two Patients in Korea. J Korean Med Sci 2020;35:e86. [Crossref] [PubMed]
- Bruno-Murtha LA, Osgood R, Lan FY, et al. SARS-CoV-2 antibody seroprevalence after the first wave among workers at a community healthcare system in the Greater Boston area. Pathog Glob Health 2021;115:331-4. [Crossref] [PubMed]
- Menachemi N, Yiannoutsos CT, Dixon BE, et al. Population Point Prevalence of SARS-CoV-2 Infection Based on a Statewide Random Sample - Indiana, April 25-29, 2020. MMWR Morb Mortal Wkly Rep 2020;69:960-4. [Crossref] [PubMed]
- Parmar H, Montovano M, Banada P, et al. RT-PCR negative COVID-19. BMC Infect Dis 2022;22:149. [Crossref] [PubMed]
- Bajema KL, Wiegand RE, Cuffe K, et al. Estimated SARS-CoV-2 Seroprevalence in the US as of September 2020. JAMA Intern Med 2021;181:450-60. [Crossref] [PubMed]
- Public Health England PD, Nuffield Department of Medicine University of Oxford, Oxford University Hospitals NHS Foundation Trust. Evaluation of sensitivity and specificity of four commercially available SARS-CoV-2 antibody immunoassays [Internet]. Oxford, England; 2020 [cited 2022 Jul 29]. Available online: https://assets.publishing.service.gov.uk/government/uploads/system/uploads/attachment_data/file/898437/Evaluation__of_sensitivity_and_specificity_of_4_commercially_available_SARS-CoV-2_antibody_immunoassays.pdf
- Mrak D, Tobudic S, Koblischke M, et al. SARS-CoV-2 vaccination in rituximab-treated patients: B cells promote humoral immune responses in the presence of T-cell-mediated immunity. Ann Rheum Dis 2021;80:1345-50. [Crossref] [PubMed]
- Guiomar R, Santos AJ, Melo AM, et al. High correlation between binding IgG (anti-RBD/S) and neutralizing antibodies against SARS-CoV-2 six months after vaccination. MedRxiv [Preprint]. 2021 [cited 2022 Jul 29]. Available online: https://www.medrxiv.org/content/10.1101/2021.12.10.21267607v1
10.1101/2021.12.10.21267607 10.1101/2021.12.10.21267607 - Kim Y, Lee JH, Ko GY, et al. Quantitative SARS-CoV-2 Spike Antibody Response in COVID-19 Patients Using Three Fully Automated Immunoassays and a Surrogate Virus Neutralization Test. Diagnostics (Basel) 2021;11:1496. [Crossref] [PubMed]
- Ekelund O, Ekblom K, Somajo S, et al. High-throughput immunoassays for SARS-CoV-2 - considerable differences in performance when comparing three methods. Infect Dis (Lond) 2021;53:805-10. [Crossref] [PubMed]
- Kahn R, Kennedy-Shaffer L, Grad YH, et al. Potential biases arising from epidemic dynamics in observational seroprotection studies. MedRxiv [Preprint]. 2020 [cited 2022 Jul 29]. Available online: https://www.medrxiv.org/content/10.1101/2020.05.02.20088765v1
10.1101/2020.05.02.20088765 10.1101/2020.05.02.20088765
Cite this article as: Cole M, Dodge MC, Duffy ER, Osterland JN, Gawel SH, Ye L, de la Cena K, Ragan EJ, Weber SE, Schechter-Perkins EM, Bouton TC, Jacobson KR, Andry C, Kataria Y. Comparison of SARS-CoV-2 anti-spike IgG and anti-nucleoprotein IgG seroprevalence amongst a pre-vaccine cohort of healthcare workers at an academic medical center in Boston, Massachusetts. J Lab Precis Med 2022;7:30.